Book Review: Intermediate Python for Data Analysis with pandas
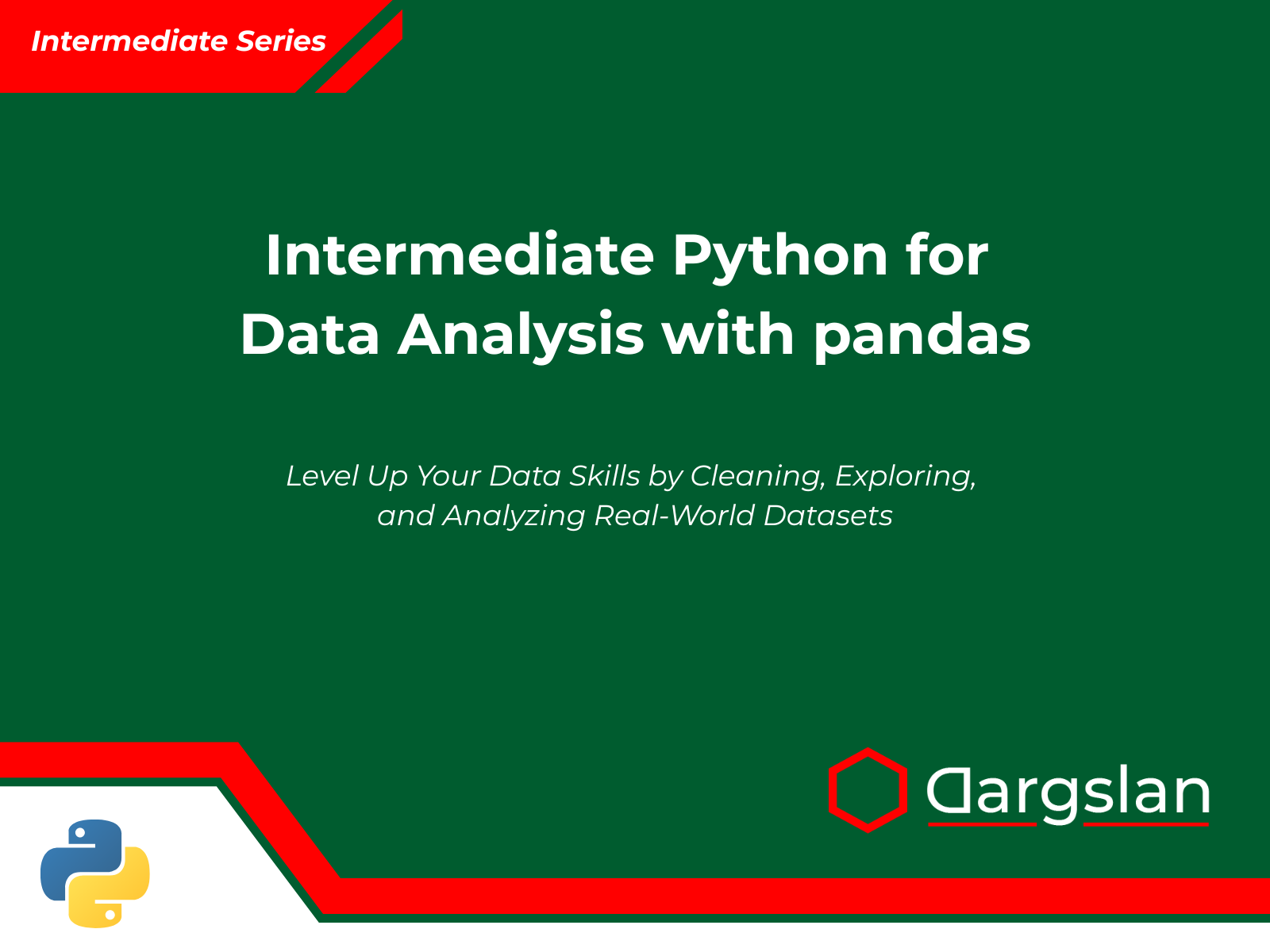
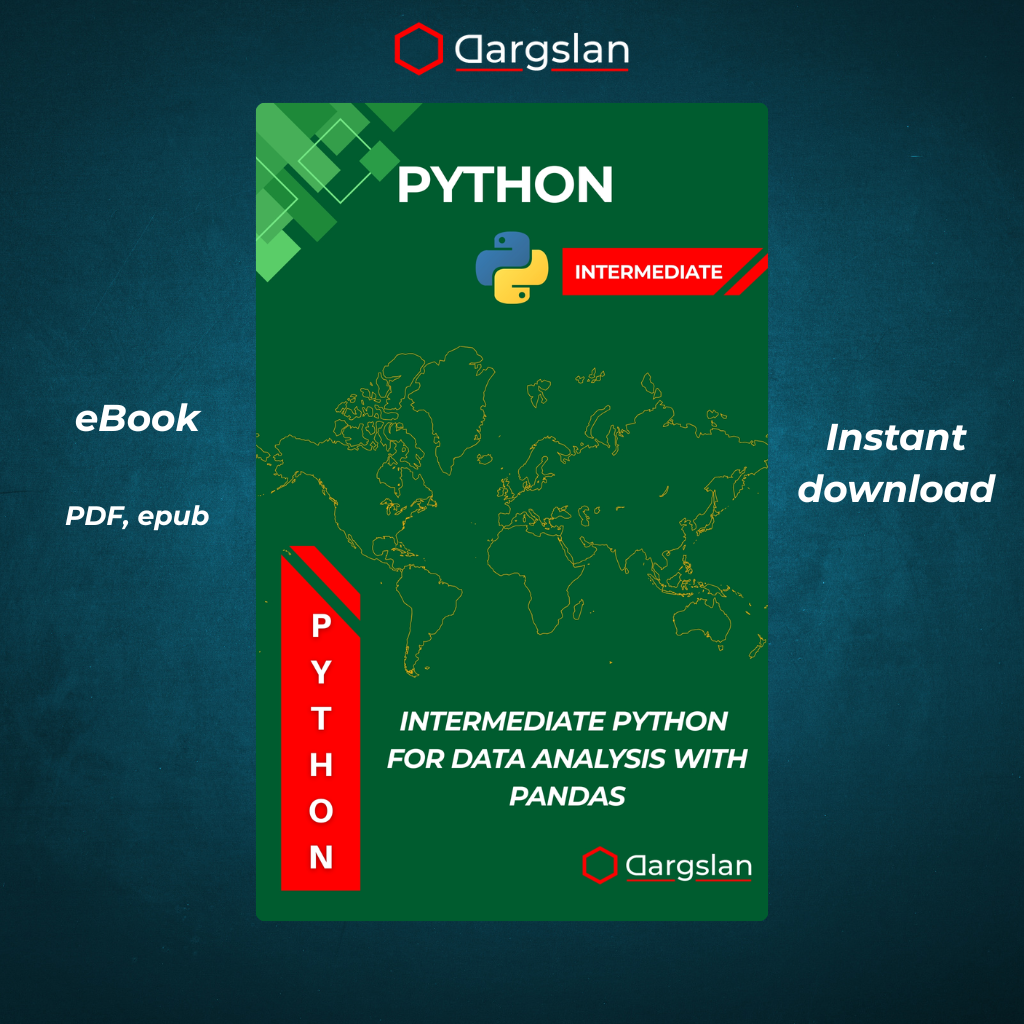
Intermediate Python for Data Analysis with pandas
Level Up Your Data Skills by Cleaning, Exploring, and Analyzing Real-World Datasets
Comprehensive Book Review: "Intermediate Python for Data Analysis with pandas"
A Detailed Guide to Mastering Python's Most Powerful Data Analysis Library
Are you ready to transform from a Python beginner to a confident data analyst? "Intermediate Python for Data Analysis with pandas" by Dargslan provides the perfect roadmap for this journey. This comprehensive book bridges the gap between basic Python programming and professional-level data analysis capabilities.
Executive Summary
"Intermediate Python for Data Analysis with pandas" is a meticulously crafted educational resource aimed at programmers and analysts who have grasped Python basics but need to develop more sophisticated data handling skills. The book excels in presenting complex pandas functionality through practical, real-world examples, making it an invaluable resource for anyone looking to advance their data analysis capabilities using Python's renowned pandas library.
With a logical progression through ten chapters and four appendices, the book covers everything from refreshing pandas fundamentals to executing complete data analysis projects. The author's approach strikes an excellent balance between theoretical explanation and hands-on practice, ensuring readers not only understand concepts but can apply them immediately to their own datasets.
Who This Book Is For
This book targets several distinct audiences:
- Python programmers looking to specialize in data analysis
- Data analysts transitioning from other tools (like Excel or R) to Python
- Business intelligence professionals seeking to automate and enhance their reporting capabilities
- Students in data science or analytics programs needing a practical pandas reference
- Self-taught data enthusiasts ready to move beyond basic pandas operations
The author assumes readers have foundational Python knowledge but structures the content to be accessible while still delivering advanced techniques and insights.
Detailed Chapter Analysis
Chapter 1: Review of pandas Basics
The book begins with a refresher on pandas fundamentals—a smart decision that ensures all readers start with a solid foundation. Rather than a tedious rehash, this chapter serves as a concise reference covering:
- DataFrame and Series object essentials
- Data importing from various sources
- Basic selection and indexing operations
- Fundamental data exploration methods
This chapter effectively bridges any knowledge gaps without overwhelming readers who are already familiar with basic pandas operations.
Chapter 2: Cleaning and Preparing Data
The journey into more advanced territory begins with perhaps the most critical aspect of any data analysis project: data cleaning. This chapter addresses the reality that real-world data is messy and teaches essential techniques for:
- Handling missing values with sophisticated strategies
- Detecting and removing duplicates
- Data type conversion and validation
- String cleaning and standardization
- Outlier detection and treatment
The author presents each technique with practical scenarios, helping readers understand not just how to clean data, but when to apply each method based on the specific context of their analysis.
Chapter 3: Filtering and Querying DataFrames
Building on the cleaned data foundation, this chapter explores the nuanced art of data filtering:
- Complex boolean indexing operations
- Chaining multiple filtering conditions
- Using the query() method for SQL-like expressions
- Applying custom functions for filtering
- Optimizing filter operations for large datasets
The examples provided demonstrate how to answer business questions through properly constructed filters, a skill essential for any analyst working with large datasets.
Chapter 4: Aggregation and Grouping
This chapter delves into one of pandas' most powerful features—its ability to group and aggregate data:
- Advanced groupby operations
- Custom aggregation functions
- Multi-level grouping strategies
- The split-apply-combine paradigm
- Pivot tables and cross-tabulations
Through carefully constructed examples, readers learn to extract meaningful summaries from complex datasets, transforming raw data into actionable insights.
Chapter 5: Merging and Joining Datasets
Few real-world analyses involve only a single dataset. This chapter covers:
- Different join types (inner, outer, left, right)
- Handling key mismatches during joins
- Concatenation vs. merging
- Performance considerations for large datasets
- Maintaining data integrity during merges
The practical examples demonstrate database-like operations within pandas, enabling readers to combine information from multiple sources seamlessly.
Chapter 6: Working with Time Series Data
Time series analysis receives dedicated attention, reflecting its importance in many data analysis contexts:
- Date and time manipulation in pandas
- Resampling time series data
- Handling time zones and frequencies
- Rolling windows and moving averages
- Time-based grouping and analysis
This chapter equips readers with techniques applicable in finance, IoT, web analytics, and numerous other fields where time-based data is prevalent.
Chapter 7: Creating New Columns and Features
Feature engineering—the art of creating new variables to enhance analysis—is covered comprehensively:
- Applying vectorized operations
- Creating derived variables
- Binning and categorization techniques
- One-hot encoding categorical variables
- Using apply(), map(), and applymap() effectively
The author demonstrates how thoughtfully created features can reveal patterns otherwise hidden in the raw data.
Chapter 8: Data Visualization with pandas and matplotlib
Data visualization is presented not as an afterthought but as an integral part of the analysis process:
- Direct plotting from pandas objects
- Customizing visualization aesthetics
- Creating multi-faceted plots
- Interactive visualization options
- Designing visualizations for different audiences
Through sample code and resulting visualizations, readers learn to create compelling visual narratives from their data.
Chapter 9: Exporting and Reporting Results
The often-overlooked step of communicating results receives proper attention:
- Exporting to various file formats
- Creating reproducible reports
- Formatting pandas output for presentation
- Automation of reporting workflows
- Best practices for data documentation
This practical chapter ensures that insights don't remain trapped in the analyst's notebook but can be effectively shared with stakeholders.
Chapter 10: Real-World Data Analysis Projects
The book culminates in comprehensive case studies that tie together all previous concepts:
- End-to-end analysis workflows
- Problem-solving approaches with real datasets
- Performance optimization for large-scale analyses
- Error handling in production environments
- Documentation and code organization
These projects serve as templates that readers can adapt to their own analytical challenges.
Valuable Appendices
The four appendices provide exceptional supplementary material:
- pandas Cheat Sheet: A quick-reference guide to the most useful pandas methods and shortcuts
- Common Errors and How to Fix Them: Troubleshooting guidance for typical pandas pitfalls
- Best Practices for Reusable Analysis Pipelines: Architectural patterns for maintainable code
- 10 Free Public Datasets to Practice With: Curated resources for continued learning
These appendices transform the book from a mere instructional text to a complete learning system.
Technical Implementation and Code Quality
The code examples throughout the book demonstrate professional-quality Python:
- Adherence to PEP 8 style guidelines
- Efficient use of pandas idioms and patterns
- Clear variable naming and documentation
- Balance between readability and performance
- Thoughtful error handling
Rather than simplistic examples, the author provides code that resembles what would be found in production environments, preparing readers for real-world implementation challenges.
Teaching Methodology
The author employs several effective pedagogical approaches:
- Progressive complexity: Each chapter builds on previous knowledge
- Real-world context: Examples drawn from genuine analysis scenarios
- Problem-solution format: Presenting analytical challenges and their pandas solutions
- Best practice emphasis: Highlighting not just what works, but what works well
- Common pitfall warnings: Alerting readers to typical mistakes and misconceptions
This methodology ensures that readers don't just memorize pandas syntax but develop genuine analytical thinking skills.
Comparison with Other Resources
In the crowded field of Python data analysis books, "Intermediate Python for Data Analysis with pandas" distinguishes itself in several ways:
- Focus on intermediate skills: Unlike many books that either cover only basics or jump too quickly to advanced topics
- Practical orientation: Emphasizes applicable skills rather than theoretical concepts
- Comprehensive pandas coverage: Explores the library's capabilities more thoroughly than general Python data science books
- Modern pandas approaches: Incorporates newer pandas methods and best practices
- End-to-end workflow perspective: Considers the entire analytical process, not just isolated techniques
The book fills an important gap between introductory pandas tutorials and highly specialized advanced texts.
What Could Be Improved
For balanced assessment, a few areas could potentially be enhanced:
- More coverage of integration with other data science libraries like scikit-learn
- Additional discussion of big data techniques when pandas reaches its memory limits
- More explicit treatment of performance optimization for very large datasets
- Expanded coverage of newer pandas features in recent releases
These minor limitations do not significantly detract from the book's overall value and may be addressed in future editions.
Who Would Benefit Most from This Book
This book provides exceptional value for several specific reader profiles:
- Data analysts transitioning to Python from other tools will find the practical approach helps them become productive quickly
- Junior data scientists will develop the pandas proficiency needed for efficient data preparation
- Business analysts will learn automation techniques that can dramatically increase their productivity
- Software developers moving into data roles will understand how to apply their coding skills to analytical problems
- Students in data-related fields will supplement their theoretical knowledge with practical implementation skills
The book's approach particularly benefits hands-on learners who prefer working through examples rather than reading abstract explanations.
Prerequisites for Readers
To get maximum value from this book, readers should have:
- Basic Python programming knowledge (variables, functions, loops, conditionals)
- Familiarity with fundamental pandas concepts (creating DataFrames, simple indexing)
- Understanding of basic data analysis concepts (mean, median, grouping)
- Access to a Python environment with pandas installed
- Ideally, some experience working with real datasets in any tool
Without this foundation, some chapters may prove challenging, though the review chapter helps mitigate this concern.
SEO-Friendly Q&A Section
Is pandas difficult to learn for intermediate Python programmers?
While pandas has a steep learning curve, "Intermediate Python for Data Analysis with pandas" makes the journey accessible by building logically from foundational concepts to advanced techniques. The book's structure helps intermediate Python programmers leverage their existing programming knowledge while gradually introducing pandas-specific paradigms.
How long does it take to become proficient in pandas for data analysis?
With dedicated study using this book, most intermediate Python programmers can become proficient in pandas within 2-3 months of part-time study. The book's hands-on approach accelerates learning by emphasizing practical application rather than rote memorization of methods.
Can pandas handle big data?
The book addresses pandas' memory limitations honestly and provides strategies for working with larger-than-memory datasets. While pandas is primarily designed for in-memory operations, the techniques taught in this book help readers optimize performance and integrate with big data tools when necessary.
Is pandas being replaced by newer Python libraries?
Despite the emergence of new libraries, pandas remains the cornerstone of Python data analysis. This book demonstrates why pandas continues to be essential, while also touching on how it integrates with newer tools in the ecosystem.
How does pandas compare to SQL for data analysis?
Chapter 3 and 5 particularly address this question, showing how pandas can replicate most SQL operations while offering greater flexibility. The book shows how pandas extends beyond SQL's capabilities while maintaining familiar query patterns for those with database backgrounds.
What real-world projects can I complete after reading this book?
The final chapter outlines several complete projects spanning different domains. After working through this book, readers will be equipped to tackle data analysis projects including financial analysis, customer behavior analysis, time series forecasting, and automated reporting systems.
Conclusion: A Valuable Investment for Data Analysts
"Intermediate Python for Data Analysis with pandas" stands out as an exceptionally practical and comprehensive guide for anyone serious about developing professional-level data analysis skills with Python. Through its thoughtful structure, real-world examples, and emphasis on best practices, the book delivers on its promise to transform readers from pandas beginners to confident data analysts.
The author's focus on practical applications ensures that readers don't just accumulate knowledge but develop applicable skills that can immediately enhance their analytical capabilities. The inclusion of complete projects and extensive supplementary materials further extends the book's value beyond its core chapters.
For intermediate Python programmers looking to specialize in data analysis, this book represents not merely an educational resource but a worthwhile investment in career-enhancing skills. In today's data-driven business environment, the capabilities taught in this book are increasingly valuable across virtually every industry.
Whether you're analyzing financial data, customer behavior, scientific measurements, or business metrics, the pandas techniques presented in this comprehensive guide will enable you to do so more efficiently, effectively, and insightfully.
About the Author
Dargslan brings practical expertise and a clear teaching approach to "Intermediate Python for Data Analysis with pandas." Through carefully structured content and realistic examples, the author demonstrates both technical proficiency with pandas and a deep understanding of real-world analytical challenges. The book reflects considerable thought about how to best guide readers from basic pandas operations to sophisticated data analysis workflows.
This review was written based on the comprehensive table of contents and preface provided for "Intermediate Python for Data Analysis with pandas" by Dargslan. The analysis reflects the book's apparent content, structure, and approach as presented in these materials.
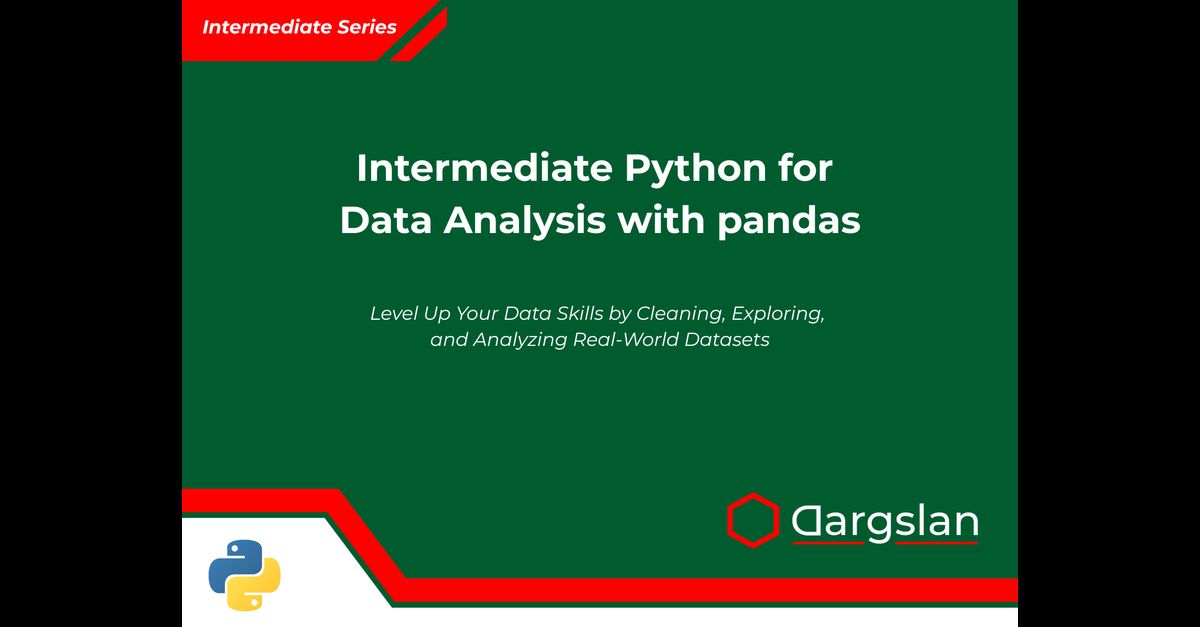
Intermediate Python for Data Analysis with pandas