Why Learn Python Programming in 2025: A Comprehensive Guide
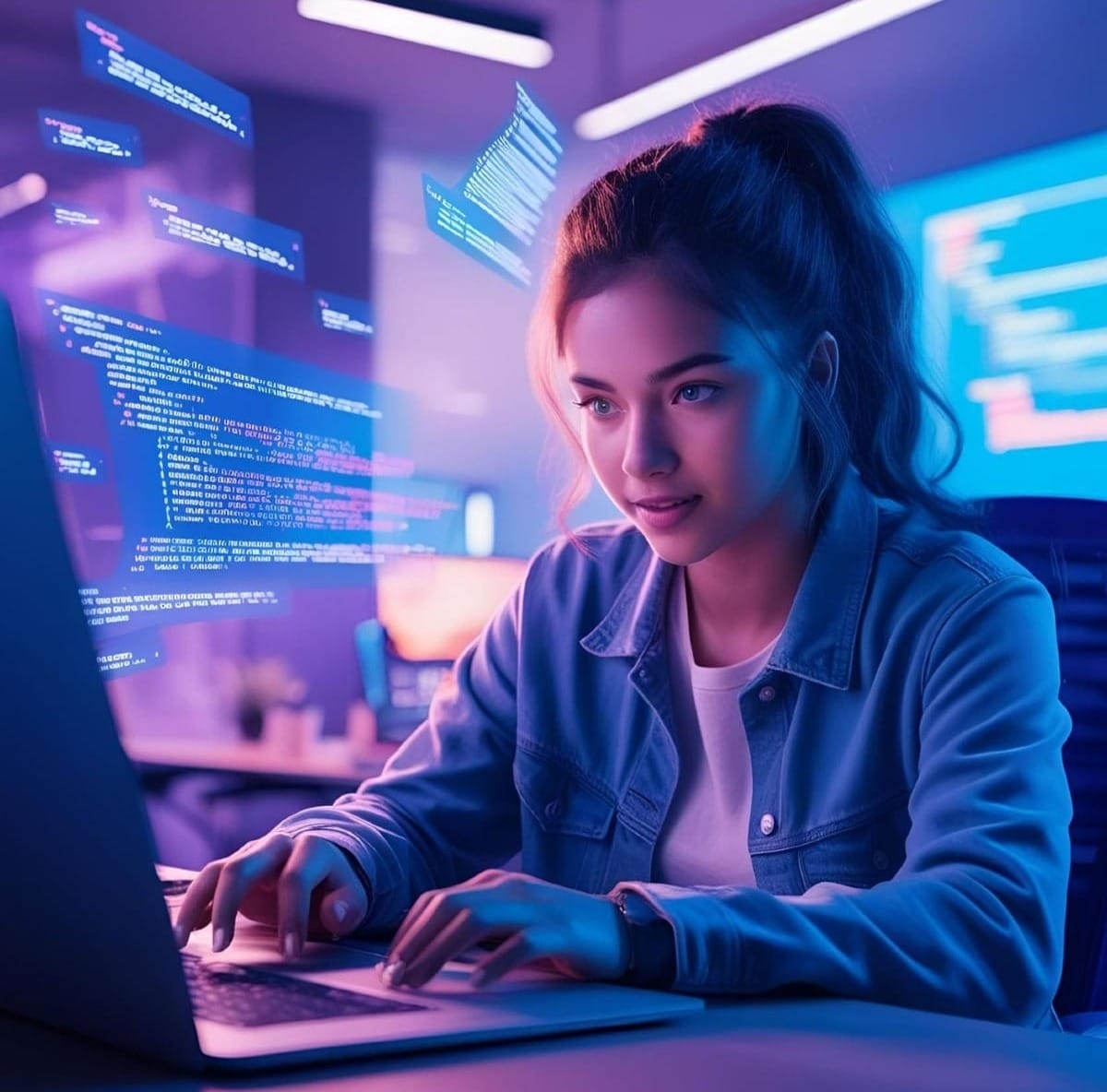
Why Learn Python Programming in 2025: A Comprehensive Guide
Table of Contents
- Introduction
- Python's Evolution: From Past to 2025
- The Python Ecosystem in 2025
- Python's Key Strengths
- Python in Data Science and AI
- Python in Web Development
- Python for Automation and DevOps
- Python in Emerging Technologies
- Python in Business and Enterprise
- Learning Python: Path to Mastery
- Python vs. Other Programming Languages
- Job Market and Career Opportunities
- Challenges and Limitations of Python
- Python Community and Support
- Future-Proofing Your Python Skills
- Conclusion
Introduction
Python has firmly established itself as one of the world's most popular programming languages, and as we approach 2025, its relevance and importance in the technology landscape continue to grow. Created by Guido van Rossum in 1991, Python's journey from a scripting language to a powerhouse in domains ranging from web development to artificial intelligence has been remarkable. Its philosophy of simplicity, readability, and versatility has attracted developers, scientists, analysts, and businesses across the globe.
This comprehensive article explores why Python remains an essential language to learn in 2025, examining its evolution, ecosystem, applications across various domains, and the opportunities it presents for both beginners and experienced programmers. We'll analyze Python's position in the job market, compare it with other popular programming languages, and provide insights into how to maximize the value of Python skills in the coming years.
Whether you're contemplating your first programming language, considering adding Python to your technical repertoire, or evaluating your organization's technology stack, this guide offers a thorough examination of Python's place in the 2025 programming landscape.
Python's Evolution: From Past to 2025
Historical Milestones
Python's 30+ year journey has been marked by continuous improvement and adaptation to changing technological landscapes:
- 1991: Python 0.9.0 released by Guido van Rossum
- 2000: Python 2.0 introduced list comprehensions and garbage collection
- 2008: Python 3.0 released, focusing on removing legacy features and improving consistency
- 2020: Python 2 reached end-of-life, consolidating the community on Python 3
- 2022-2023: Rapid release schedule of Python 3.10 and 3.11 with significant performance improvements
- 2024-2025: Anticipated releases focusing on enhanced performance, better concurrency models, and refined typing systems
Recent Developments
Python's evolution in the years leading up to 2025 has been characterized by:
-
Performance Enhancements: The Python core team has placed significant emphasis on speed, with Python 3.11 already delivering 10-60% faster performance than 3.10. By 2025, we anticipate further improvements, potentially narrowing the gap with compiled languages for many use cases.
-
Type Hinting Maturation: Since the introduction of type hints in PEP 484 (Python 3.5), Python's typing system has evolved significantly. By 2025, we expect type checking to be more deeply integrated into the development workflow, with enhanced IDE support and improved static analysis tools.
-
Concurrency Model Improvements: Python's asynchronous programming model has matured through asyncio and related libraries. The language continues to evolve better patterns for handling concurrency, addressing one of its historical limitations.
-
Pattern Matching: Introduced in Python 3.10, pattern matching brought more expressive ways to handle complex data structures. By 2025, this paradigm will be fully integrated into Python's standard practices.
The Python Enhancement Proposal (PEP) Process
Python's evolution is guided by its PEP process, which has maintained a balance between innovation and stability. Some recent influential PEPs that will shape Python's landscape in 2025 include:
- PEP 621: Standardizing project metadata in pyproject.toml
- PEP 660/662: Editable installs for pip and packaging improvements
- PEP 673: Self type for improved class method typing
- PEP 695: Type parameter syntax for typed classes and functions (Python 3.12)
The structured PEP process ensures Python continues to evolve in a manner that respects its core philosophy while adapting to modern development needs.
Python's Core Philosophy
Python's enduring success stems from its adherence to a set of principles outlined in "The Zen of Python" (PEP 20). These principles, including "Readability counts" and "Simple is better than complex," continue to guide Python's design choices, ensuring that even as the language evolves, it maintains its fundamental character of clarity and readability.
The Python Ecosystem in 2025
Package Landscape
By 2025, Python's package ecosystem has grown exponentially from its already impressive size of over 400,000 packages on PyPI (Python Package Index) in 2023. This rich ecosystem spans virtually every domain of computing and continues to be one of Python's most significant advantages.
Key Categories in Python's Package Ecosystem:
Category | Description | Notable Packages | Trends in 2025 |
---|---|---|---|
Data Science | Libraries for data analysis, visualization, and machine learning | NumPy, Pandas, Matplotlib, SciPy | Enhanced GPU integration, distributed computing capabilities |
Web Development | Frameworks and tools for building web applications | Django, Flask, FastAPI, Starlette | Improved performance, WebAssembly integration, enhanced async capabilities |
DevOps and Infrastructure | Tools for automation, deployment, and monitoring | Ansible, Fabric, Pulumi, Locust | Expanded cloud-native integrations, enhanced security features |
Testing and Quality | Frameworks for testing, linting, and quality assurance | pytest, unittest, pylint, mypy | More sophisticated static analysis, enhanced property-based testing |
Scientific Computing | Specialized tools for scientific domains | SciPy, Biopython, AstroPy, SimPy | Deeper integration with domain-specific hardware, improved simulation capabilities |
AI and Machine Learning | Frameworks for building and deploying AI/ML models | TensorFlow, PyTorch, Scikit-learn, Hugging Face | Advanced multimodal models, improved explainability tools, edge deployment |
Development Tools and IDEs
The Python development experience in 2025 is characterized by sophisticated tooling that combines ease of use with powerful capabilities:
-
Integrated Development Environments (IDEs):
- PyCharm: Continues to lead for professional developers with its comprehensive feature set
- VS Code: Has gained significant market share with its lightweight yet powerful Python extension
- Jupyter Evolution: Advanced from notebooks to full development environments with improved collaboration features
- AI-Enhanced IDEs: Integration of AI coding assistants that understand Python's idioms and best practices
-
Build and Dependency Management:
- Poetry: Widely adopted as the standard for Python dependency management
- PDM: Gained popularity for its PEP 582 support and performance
- Containerization Integration: Seamless development workflows with Docker and Kubernetes
-
Performance and Debugging Tools:
- Advanced profiling tools with visual interfaces
- Time-travel debugging capabilities
- Memory analyzers for optimizing resource usage
Package Management and Virtual Environments
The Python packaging ecosystem has undergone significant standardization by 2025:
-
Unified Standards:
- Widespread adoption of
pyproject.toml
as the standard configuration file - Consistent build system interfaces across the ecosystem
- Widespread adoption of
-
Virtual Environment Management:
- Simplified virtual environment workflows with integrated tool chains
- Better integration with system-level package managers on various operating systems
-
Distribution and Deployment:
- Streamlined paths from development to production
- Enhanced binary distribution options reducing dependency conflicts
- Improved support for mobile and edge computing environments
Python's Key Strengths
Readability and Ease of Learning
Python's syntax emphasizes readability through its use of whitespace indentation and minimalist approach to syntax elements. This design philosophy makes Python particularly accessible to beginners while remaining pleasant for experienced developers.
# Example of Python's readable syntax
def calculate_average(numbers):
"""Calculate the average of a list of numbers."""
if not numbers:
return 0
return sum(numbers) / len(numbers)
# Using list comprehension - a powerful yet readable feature
squares = [x**2 for x in range(10) if x % 2 == 0]
The readability advantage translates to:
- Faster onboarding for new team members
- Reduced maintenance costs
- Fewer bugs and easier debugging
- Better collaboration between technical and non-technical stakeholders
By 2025, Python's commitment to readability has been further enhanced through language features that reduce boilerplate while maintaining clarity.
Versatility Across Domains
Python's remarkable versatility allows it to excel in numerous domains, making it a valuable skill regardless of career path. This versatility stems from:
- Extensibility: Python's C API allows integration with high-performance libraries
- Adaptable Paradigms: Support for procedural, object-oriented, and functional programming
- Domain-Specific Libraries: Specialized tools for virtually every field
- Cross-Platform Compatibility: Runs on all major operating systems and many embedded platforms
This versatility means Python skills transfer effectively between domains, providing career flexibility and allowing organizations to maintain consistent technology stacks across different applications.
Extensive Standard Library
The Python Standard Library follows the "batteries included" philosophy, providing built-in support for many common programming tasks:
- File I/O and filesystem operations
- Data structures and algorithms
- Network communication protocols
- Concurrent programming tools
- Testing frameworks
- Data serialization formats
- And much more
This comprehensive foundation reduces dependency on external libraries for many basic tasks, improving maintainability and security. By 2025, the standard library has continued to evolve, incorporating modern practices while maintaining backward compatibility.
Strong Community Support
Python's community is one of its greatest assets, characterized by:
- Size and Diversity: Millions of developers worldwide across various industries
- Knowledge Sharing: Extensive documentation, tutorials, forums, and educational resources
- Corporate Backing: Major companies like Google, Microsoft, and Amazon invest in Python's ecosystem
- Open Governance: Transparent decision-making processes through the Python Software Foundation
This robust community ensures that Python developers have access to support, resources, and collaborative opportunities unmatched by many other languages.
Python in Data Science and AI
Python's Dominance in Data Analysis
Python has solidified its position as the preeminent language for data analysis and manipulation, with several key libraries forming the foundation of its data science ecosystem:
Core Data Science Stack
Library | Primary Function | 2025 Enhancements |
---|---|---|
NumPy | Numerical computing with multi-dimensional arrays | Enhanced GPU/TPU integration, improved memory efficiency |
Pandas | Data manipulation and analysis | Distributed dataframes, improved performance for large datasets |
Matplotlib/Seaborn | Data visualization | Interactive web visualizations, AR/VR data exploration capabilities |
SciPy | Scientific computing algorithms | Expanded algorithm collection, better parallelization |
Statsmodels | Statistical models and tests | Enhanced Bayesian methods, causal inference tools |
The integration between these libraries has become seamless by 2025, with consistent APIs and data exchange formats enabling fluid workflows. Performance improvements have addressed many historical bottlenecks, making Python competitive with specialized data processing languages for many common tasks.
Machine Learning Frameworks
Python's machine learning ecosystem continues to thrive in 2025, with frameworks that balance ease of use and cutting-edge capabilities:
-
Scikit-learn: Remains the standard for traditional machine learning algorithms with an expanded library of techniques and improved scalability.
-
TensorFlow and PyTorch: These deep learning frameworks have evolved significantly:
- Enhanced model development experience through higher-level APIs
- Improved deployment options across heterogeneous computing environments
- Better integration with model monitoring and explainability tools
- Specialized extensions for emerging AI architectures
-
AutoML Platforms: Python-based automated machine learning tools have matured, democratizing access to advanced modeling techniques:
- Automated feature engineering and selection
- Neural architecture search
- Hyperparameter optimization with sophisticated techniques
- Model selection and ensemble creation
Large Language Models (LLMs) and AI Tools
By 2025, Python has become the primary interface language for working with large language models and generative AI:
-
Specialized Frameworks: Libraries dedicated to prompt engineering, model fine-tuning, and deployment of large language models have proliferated.
-
Ethical AI Tools: Python packages for bias detection, model fairness assessment, and explainability have become standard components of the AI development workflow.
-
Multimodal AI: Python libraries now support seamless work across text, image, audio, and video data for building comprehensive AI systems.
-
Domain-Specific AI: Specialized packages have emerged for fields like healthcare, finance, and scientific research, with pre-built components addressing domain-specific challenges.
# Example of working with a multimodal model in Python (2025)
import multimodal_ai as mm
# Load a pre-trained model with ethical constraints
model = mm.load_model("general-purpose-v4", ethical_constraints=True)
# Process mixed data types
analysis = model.analyze({
"text": customer_feedback,
"image": product_photo,
"time_series": usage_data
})
# Generate insights with uncertainty estimates
insights = analysis.generate_insights(confidence_threshold=0.85)
Data Engineering and Big Data
Python's role in data engineering has expanded by 2025, with improved tools for handling large-scale data processing:
-
Distributed Computing: Enhanced libraries for Apache Spark, Dask, and Ray provide intuitive interfaces for distributed computing.
-
Stream Processing: Python frameworks for real-time data processing have matured, with native support for event-driven architectures.
-
Data Pipelines: Python-based orchestration tools create reproducible, monitored data workflows from ingestion to deployment.
-
Data Quality and Governance: Specialized libraries for ensuring data quality, lineage tracking, and regulatory compliance have become essential parts of the Python data ecosystem.
Python in Web Development
Web Frameworks Evolution
Python's web development ecosystem has evolved significantly by 2025, offering solutions for everything from simple APIs to complex web applications:
Major Web Frameworks
Framework | Type | Key Features | Best For |
---|---|---|---|
Django | Full-stack | Admin interface, ORM, security features | Complex applications, content sites |
Flask | Microframework | Lightweight, extensible, minimalist | APIs, microservices, custom needs |
FastAPI | API-focused | Async support, automatic docs, validation | High-performance APIs, microservices |
Starlette | ASGI framework | High performance, WebSocket support | Real-time applications, high throughput services |
Quart | Flask-compatible | Async version of Flask API | Migrating Flask apps to async |
These frameworks have continued to evolve their developer experience while maintaining their distinct philosophies:
-
Django: Has modernized its frontend integration approach while maintaining its "batteries-included" philosophy, with improved async support and enhanced security features.
-
Flask: Continues to emphasize simplicity and extension, with a mature ecosystem of plugins and better integration with modern frontend frameworks.
-
FastAPI: Has grown into a comprehensive ecosystem with expanded validation capabilities, sophisticated dependency injection, and enhanced security features.
Asynchronous Web Development
Asynchronous programming has become the standard approach for Python web development by 2025:
-
ASGI Standard: The Asynchronous Server Gateway Interface has matured with widespread server support and middleware options.
-
Performance Improvements: Async Python web servers now achieve performance comparable to Node.js and Go for many common scenarios.
-
Development Experience: Async debugging tools, profiling, and testing frameworks have evolved to make async development more accessible.
# Example of a modern async API endpoint in Python (2025)
from fastapi import FastAPI, Depends, HTTPException
from sqlalchemy.ext.asyncio import AsyncSession
app = FastAPI()
@app.get("/users/{user_id}/recommendations")
async def get_recommendations(
user_id: int,
session: AsyncSession = Depends(get_session),
recommendation_engine = Depends(get_recommendation_engine)
):
user = await session.get(User, user_id)
if not user:
raise HTTPException(status_code=404, detail="User not found")
# Perform concurrent operations
recommendations = await recommendation_engine.get_for_user(user)
user_stats = await session.execute(select(UserStats).where(UserStats.user_id == user_id))
# Process results
return {
"recommendations": recommendations,
"user_stats": user_stats.first()
}
Python in Full-Stack Development
Modern Python web development in 2025 embraces a variety of approaches to frontend integration:
-
API-First Development: Python backends serving JSON APIs consumed by JavaScript frontends remains popular, with improved tooling for API design, documentation, and testing.
-
Server-Side Rendering: Enhanced template engines and frameworks support hybrid rendering approaches that balance SEO needs with interactive experiences.
-
WebAssembly Integration: Python can now compile to WebAssembly more efficiently, enabling Python code to run directly in browsers with performance approaching JavaScript.
-
Python Full-Stack Frameworks: A new generation of frameworks inspired by Ruby on Rails and Laravel has emerged, offering streamlined full-stack development experiences.
Real-Time Web Applications
Python's capabilities for real-time web applications have expanded significantly:
-
WebSocket Support: Native WebSocket handling in async frameworks enables efficient bidirectional communication.
-
Event-Driven Architectures: Integration with message brokers and event systems allows scalable real-time features.
-
Push Notifications: Standardized approaches for web push, mobile notifications, and real-time alerts have emerged within the Python ecosystem.
Python for Automation and DevOps
Infrastructure as Code
Python has become a leading language for infrastructure as code (IaC) implementations, offering a powerful balance of readability and flexibility:
-
Cloud Provider SDKs: All major cloud platforms provide comprehensive Python SDKs that have evolved to include type hints, better documentation, and simplified authentication patterns.
-
IaC Frameworks:
- Pulumi: The Python SDK offers programmatic infrastructure definition with full access to Python's capabilities
- AWS CDK: Python support for the Cloud Development Kit has matured with comprehensive component libraries
- Terraform: Enhanced Python integration through improved bindings and generators
-
Configuration Management:
- Ansible: Continues to leverage Python for both its core and for custom modules
- SaltStack: Python-based configuration management with enhanced security features
- Custom Solutions: Python's flexibility enables organization-specific tools
# Example of infrastructure as code with Python (2025)
import pulumi
import pulumi_aws as aws
# Create a VPC and subnets
vpc = aws.ec2.Vpc("app-vpc",
cidr_block="10.0.0.0/16",
enable_dns_hostnames=True,
tags={"Name": "application-vpc"}
)
# Create subnets across availability zones
public_subnets = []
for i, az in enumerate(aws.get_availability_zones().names[:3]):
subnet = aws.ec2.Subnet(f"public-subnet-{i}",
vpc_id=vpc.id,
cidr_block=f"10.0.{i}.0/24",
availability_zone=az,
map_public_ip_on_launch=True,
tags={"Name": f"public-subnet-{az}"}
)
public_subnets.append(subnet)
# Set up container infrastructure
cluster = aws.ecs.Cluster("app-cluster",
setting=[{"name": "containerInsights", "value": "enabled"}]
)
# Export key outputs
pulumi.export('vpc_id', vpc.id)
pulumi.export('public_subnet_ids', [subnet.id for subnet in public_subnets])
pulumi.export('cluster_name', cluster.name)
Automation and Scripting
Python's role in automation continues to expand across various domains:
-
System Administration: Python scripts have largely replaced shell scripts for complex automation tasks, offering better error handling, maintainability, and cross-platform compatibility.
-
Network Automation: Python has become the de facto standard for network configuration and management, with libraries for all major vendors and protocols.
-
Workflow Automation: Task orchestration tools with Python interfaces enable complex business process automation:
- Airflow: Scheduling and monitoring workflows
- Prefect: Modern dataflow management
- Luigi: Pipeline building and dependency resolution
-
RPA (Robotic Process Automation): Python-based RPA solutions have matured, offering alternatives to proprietary tools for business process automation.
Continuous Integration and Deployment
Python's ecosystem includes powerful tools for CI/CD processes:
-
Testing Frameworks:
- pytest: Continues to dominate with extended plugins and parallelization
- Robot Framework: For acceptance testing and RPA
- Behave/Cucumber: For behavior-driven development
-
Build and Package Tools:
- Standardized build systems via PEP 517/518
- Improved binary packaging and distribution
- Container integration tools
-
Deployment Automation:
- Blue/green deployment orchestration
- Canary release management
- Configuration validation and security checking
Monitoring and Observability
Python plays a crucial role in the observability stack:
-
Instrumentation Libraries:
- OpenTelemetry integration for tracing, metrics, and logs
- Application Performance Monitoring (APM) agents
- Custom monitoring solutions
-
Data Processing for Monitoring:
- Log analysis and aggregation
- Anomaly detection pipelines
- Alert correlation and management
-
Dashboarding and Visualization:
- Python-based web dashboards
- Notebook integrations for exploratory analysis
- Custom reporting tools
Python in Emerging Technologies
Internet of Things (IoT)
Python has established itself as a key language in the IoT ecosystem by 2025:
-
MicroPython and CircuitPython: These Python implementations for microcontrollers have expanded their hardware support and capabilities:
- Enhanced memory efficiency
- Better power management features
- Broader peripheral support
- Wireless communication libraries
-
Edge Computing: Python serves as the bridge between edge devices and cloud infrastructure:
- Optimized ML model deployment on edge devices
- Data preprocessing and filtering at the edge
- Secure communication protocols
-
IoT Platforms: Major IoT platforms provide Python SDKs and integration tools:
- Simplified device registration and management
- Standardized approaches to device update and maintenance
- Analytics integrations
# Example of IoT device code with MicroPython (2025)
import machine
import network
import time
import mqtt
from sensors import EnvironmentalSensor
from machine import low_power_mode
# Configure device
led = machine.Pin(2, machine.Pin.OUT)
sensor = EnvironmentalSensor(i2c=machine.I2C(0))
# Connect to network
wifi = network.WLAN(network.STA_IF)
wifi.connect('NetworkName', 'password')
while not wifi.isconnected():
time.sleep(0.1)
# Setup MQTT client
client = mqtt.MQTTClient("device123", "mqtt.example.com")
client.connect()
# Main operation loop
while True:
# Collect data
readings = sensor.read_all()
# Publish data
client.publish("sensors/env", json.dumps(readings))
led.on()
time.sleep(0.2)
led.off()
# Go to low power mode between readings
low_power_mode(60) # Sleep for 60 seconds
Quantum Computing
Python has become the primary language for quantum computing experimentation and development:
-
Quantum Frameworks:
- Qiskit: IBM's framework has expanded with specialized modules for various quantum computing domains
- Cirq: Google's library has matured with better simulator performance and hardware access
- PennyLane: Quantum machine learning library with enhanced gradient calculation methods
- Unified interfaces: Standards have emerged for interoperability between different quantum systems
-
Quantum Education: Python's accessibility has made it central to quantum computing education:
- Interactive tutorials and simulations
- Visualizations of quantum states and operations
- Simplified abstractions for beginners
-
Hybrid Classical-Quantum Computing: Python serves as the bridge between classical and quantum systems:
- Optimization of algorithm partitioning
- Resource management across computing paradigms
- Result verification and validation
Blockchain and Cryptography
Python's role in blockchain development has grown significantly:
-
Blockchain Development:
- Smart contract development and testing
- Blockchain network simulation and analysis
- Node implementation and management
-
Cryptographic Tools:
- Advanced cryptographic libraries with better performance
- Zero-knowledge proof implementations
- Key management systems
-
Decentralized Applications (DApps):
- Frameworks for building DApp backends
- Integration with distributed storage systems
- Identity and access management solutions
Robotics and Computer Vision
Python continues to excel in robotics and computer vision applications:
-
Robotics Frameworks:
- ROS (Robot Operating System): Python interfaces with improved performance and tooling
- PyRobot: Simplified abstractions for common robotics tasks
- Integration libraries: For major robotics platforms and manufacturers
-
Computer Vision:
- OpenCV: Enhanced with deep learning integration and improved performance
- Specialized libraries: For medical imaging, satellite imagery, and industrial inspection
- 3D vision: Libraries for point cloud processing, SLAM, and spatial mapping
-
Simulation Environments:
- Python interfaces to physics engines and virtual environments
- Digital twin implementations
- Training environments for reinforcement learning
Python in Business and Enterprise
Corporate Adoption Trends
Python's adoption in enterprise environments has accelerated by 2025, driven by several factors:
-
Cross-Functional Utility: Python serves multiple business functions:
- Data analysis and business intelligence
- Process automation and efficiency
- Application development and integration
- AI/ML implementation
-
Technology Modernization: Organizations leveraging Python for:
- Legacy system modernization
- Cloud migration
- Digital transformation initiatives
- Technical debt reduction
-
Competitive Advantage: Companies using Python to:
- Accelerate innovation cycles
- Improve time-to-market
- Enhance customer experiences through data-driven approaches
- Build internal tools and capabilities
Enterprise Adoption Metrics (2023-2025)
Industry | Adoption Growth | Primary Use Cases | Key Drivers |
---|---|---|---|
Financial Services | +42% | Risk modeling, fraud detection, automation | Regulatory requirements, cost reduction |
Healthcare | +58% | Patient data analysis, medical imaging, operational efficiency | Precision medicine, cost containment |
Manufacturing | +63% | IoT integration, predictive maintenance, supply chain optimization | Industry 4.0 initiatives, competitive pressures |
Retail | +45% | Customer analytics, inventory optimization, personalization | E-commerce growth, margin pressure |
Public Sector | +38% | Citizen services, resource allocation, transparency initiatives | Modernization mandates, efficiency requirements |
Return on Investment (ROI)
Organizations implementing Python-based solutions have documented substantial ROI across multiple dimensions:
-
Development Efficiency:
- 20-40% reduction in development time compared to traditional languages
- Lower maintenance costs due to code readability and simplicity
- Faster onboarding of new developers (typically 30-50% faster)
-
Operational Benefits:
- Automation of manual processes yields 40-70% time savings
- Data analysis capabilities improve decision quality by 15-25%
- Systems integration becomes 30-50% less costly
-
Strategic Advantages:
- Faster innovation cycles (25-40% improvement)
- Greater business agility and responsiveness
- Better talent attraction and retention
Enterprise Python Solutions
The Python ecosystem offers numerous enterprise-grade solutions:
-
Security and Compliance:
- Static analysis tools for vulnerability detection
- Supply chain security monitoring
- Compliance automation frameworks
-
Performance Optimization:
- Profiling and monitoring tools
- Just-in-Time (JIT) compilation options
- Distributed computing frameworks
-
Integration Capabilities:
- Enterprise service bus integrations
- Legacy system connectors
- API management tools
-
Support and Maintenance:
- Commercial support options from major vendors
- Long-term support distributions
- Enterprise-focused IDE features
Case Studies: Enterprise Python Success Stories
Financial Services: Global Bank Transformation
A global banking institution migrated its risk assessment systems from legacy C++ applications to a Python-based platform, resulting in:
- 65% faster model development cycle
- 40% reduction in code base size
- $12M annual savings in maintenance costs
- Improved regulatory compliance through better transparency
Healthcare: Patient Care Optimization
A hospital network implemented Python-based predictive analytics for patient care management:
- 28% reduction in readmission rates
- 15% improvement in resource allocation efficiency
- Integration of previously siloed data systems
- Enhanced clinical decision support
Manufacturing: Smart Factory Implementation
A manufacturing conglomerate used Python for IoT integration and production optimization:
- Real-time quality control improved yield by 12%
- Predictive maintenance reduced downtime by 31%
- Energy consumption optimization saved 18% in utility costs
- Unified data platform provided enterprise-wide visibility
Learning Python: Path to Mastery
Getting Started with Python
For beginners, Python offers a gentle learning curve with abundant resources:
-
Installation and Setup:
- Simplified installation through package managers
- Online environments like Google Colab, Replit, and Jupyter environments
- Development environment recommendations based on intended use cases
-
Foundational Concepts:
- Basic syntax and data types
- Control structures and functions
- Object-oriented programming fundamentals
- Modules and packages
-
Recommended Learning Resources for Beginners:
- Interactive platforms: Codecademy, DataCamp, Python.org tutorials
- Books: "Python Crash Course," "Automate the Boring Stuff with Python"
- Video courses: Coursera's "Python for Everybody," edX's "Introduction to Python Programming"
- Community learning: Local Python user groups, online forums like r/learnpython
Intermediate Python Development
As learners progress, they can focus on more advanced concepts:
-
Advanced Language Features:
- Decorators, generators, and context managers
- Type annotations and static type checking
- Concurrency models: threading, multiprocessing, and asyncio
- Metaprogramming and reflection
-
Software Design and Architecture:
- Design patterns in Python
- Testing strategies and frameworks
- Package structure and distribution
- Documentation best practices
-
Specialized Domain Knowledge:
- Web development fundamentals
- Data analysis basics
- Automation principles
- API design and consumption
Specialization Paths
By 2025, clear specialization tracks have emerged within the Python ecosystem:
-
Data Science and ML Track:
- Foundational: NumPy, Pandas, Matplotlib
- Machine Learning: Scikit-learn, feature engineering
- Deep Learning: TensorFlow/PyTorch fundamentals
- MLOps: Model deployment and monitoring
-
Web Development Track:
- Backend: Django/Flask/FastAPI
- Database: SQL/ORM/NoSQL integration
- API Design: RESTful and GraphQL services
- Full-stack: Frontend integration and deployment
-
Automation and DevOps Track:
- Systems: OS interaction, process management
- Networking: Protocols and services
- Infrastructure: Cloud providers and services
- CI/CD: Testing and deployment pipelines
-
Enterprise Development Track:
- Integration: Enterprise systems and databases
- Security: Secure coding practices
- Performance: Optimization and scaling
- Compliance: Regulatory and standard adherence
Certification and Validation
The Python certification landscape has matured by 2025:
-
Recognized Certifications:
- PCEP/PCAP/PCPP (Python Institute): Foundational Python knowledge
- Domain-specific certifications: Data science, web development, cybersecurity
- Vendor certifications: Cloud provider Python specializations
-
Portfolio Development:
- Open source contribution strategies
- Personal project showcases
- Hackathon and competition participation
-
Continuous Learning Approaches:
- Structured learning paths on platforms like Pluralsight and LinkedIn Learning
- Specialized communities for advanced topics
- Mentorship and coaching options
Python vs. Other Programming Languages
Comparative Analysis
Python exists within a diverse programming language ecosystem, each with strengths and weaknesses. Here's how Python compares to other major languages in 2025:
Language Comparison Table
Language | Strengths | Weaknesses | Best Use Cases | Python Advantage |
---|---|---|---|---|
JavaScript/TypeScript | Browser execution, vast ecosystem, full-stack development | Less suitable for heavy computation, package management complexity | Web development, mobile apps (React Native) | Cleaner syntax, better for data analysis, more predictable behavior |
Java | Enterprise adoption, strong typing, performance | Verbose, slower development cycle | Large enterprise systems, Android development | Faster development, better for prototyping, easier maintenance |
C/C++ | Speed, memory control, hardware access | Complexity, safety issues, steep learning curve | System programming, embedded systems, game engines | Dramatically faster development, safer defaults, extensive libraries |
Go | Concurrency, compilation, performance | Less expressive, fewer libraries | Microservices, distributed systems, cloud tools | More libraries, better for data science, easier learning curve |
Rust | Memory safety, performance, concurrency | Complexity, steep learning curve | Systems programming, performance-critical applications | Faster development cycle, broader library ecosystem, more accessible |
R | Statistical computing, visualization | Limited general-purpose features | Statistical analysis, research, visualization | Better general-purpose capabilities, more integration options |
Julia | Numerical computing performance, scientific focus | Smaller ecosystem, less enterprise adoption | Scientific computing, numerical simulation | Broader ecosystem, more libraries, better general-purpose support |
Performance Benchmarks
Python's performance has improved significantly, though trade-offs remain:
-
Compute-Intensive Tasks:
- Python with optimized libraries (NumPy, TensorFlow) approaches C++ performance for vectorized operations
- Just-in-Time compilation options narrow the gap for algorithmic code
- Still lags behind C/C++/Rust for low-level system operations
-
I/O and Network Operations:
- Async Python performs comparably to Go and Node.js for many web workloads
- GIL limitations still impact certain multi-threaded scenarios
- Excellent performance for I/O-bound and event-driven architectures
-
Memory Usage:
- Higher baseline memory usage than C/C++/Rust
- Improved memory efficiency in Python 3.11+ reduces this gap
- Tools for memory profiling and optimization have matured
When to Choose Python (and When Not To)
Python is particularly well-suited for:
-
Rapid Development Scenarios:
- Prototyping and proof-of-concept work
- Internal tools and automation
- Data exploration and analysis
- MVPs and early-stage startups
-
Data-Intensive Applications:
- Analytics and business intelligence
- Machine learning and AI development
- Scientific computing and research
- ETL pipelines and data engineering
-
Integration and Glue Code:
- System integration
- API development and consumption
- Automation of cross-system workflows
- Plugin development
Python may not be the best choice for:
-
Performance-Critical Systems:
- Real-time trading platforms
- Game engines and graphics processing
- Embedded systems with severe resource constraints
- Real-time operating systems
-
Mobile Application Development:
- Native mobile apps (though Python frameworks exist)
- Performance-sensitive mobile games
- Low-level device access
-
Safety-Critical Systems:
- Medical devices with certification requirements
- Aviation and automotive control systems
- Systems requiring formal verification
Job Market and Career Opportunities
Demand for Python Skills
The job market for Python developers has shown consistent growth through 2025:
-
Job Posting Trends:
- Python-related job postings have increased by approximately 25% annually
- Python has maintained its position in the top 3 most requested programming languages
- Python is increasingly listed as a required or preferred skill in non-developer roles
-
Industry Distribution:
- Technology: 32% of Python jobs
- Finance and Banking: 18%
- Healthcare and Life Sciences: 14%
- Manufacturing and Industry: 12%
- Other sectors (education, retail, government): 24%
-
Role Expansion:
- Python skills are now required beyond traditional development roles:
- Data analysts and business analysts
- Marketing technology specialists
- Product managers
- Financial analysts
- Scientific researchers
- Python skills are now required beyond traditional development roles:
Salary Trends
Python proficiency continues to command competitive compensation:
Python Developer Salary Ranges by Experience (2025)
Experience Level | US Average ($) | EU Average (€) | Asia Average ($) |
---|---|---|---|
Entry-level (0-2 years) | 85,000 - 110,000 | 45,000 - 65,000 | 25,000 - 40,000 |
Mid-level (3-5 years) | 110,000 - 145,000 | 65,000 - 95,000 | 40,000 - 70,000 |
Senior (6-9 years) | 145,000 - 180,000 | 95,000 - 120,000 | 70,000 - 100,000 |
Lead/Architect (10+ years) | 180,000 - 250,000+ | 120,000 - 180,000+ | 100,000 - 150,000+ |
Salary premiums exist for specialized expertise:
- Machine Learning/AI: +15-25%
- Cloud/DevOps: +10-20%
- Financial domain knowledge: +15-30%
- Healthcare domain knowledge: +10-25%
In-Demand Python Specializations
Certain Python specializations command particular market value:
-
Machine Learning and AI:
- Model development and deployment
- Natural language processing
- Computer vision
- Generative AI and LLMs
-
Data Engineering:
- Big data processing frameworks
- Data pipeline construction
- ETL process development
- Data quality and governance
-
Cloud and DevOps:
- Infrastructure as Code
- Cloud-native application development
- Monitoring and observability
- Container orchestration
-
Web API Development:
- Microservices architecture
- RESTful and GraphQL APIs
- Authentication and security
- Performance optimization
Career Progression Paths
Python expertise enables multiple career trajectories:
-
Technical Track:
- Junior Developer → Senior Developer → Tech Lead → Architect
- Focus on deepening Python expertise and system design skills
-
Specialization Track:
- Python Developer → ML Engineer → AI Specialist → AI Architect
- Emphasis on domain-specific knowledge and advanced techniques
-
Leadership Track:
- Developer → Team Lead → Engineering Manager → CTO
- Building management skills while maintaining technical foundation
-
Entrepreneurial Track:
- Developer → Technical Co-founder → CTO/CEO
- Using Python for rapid prototyping and product development
Challenges and Limitations of Python
Performance Considerations
While Python's performance has improved, certain limitations remain:
-
Global Interpreter Lock (GIL):
- Continues to limit true parallelism in CPU-bound tasks
- Workarounds include multiprocessing and C extensions
- Proposals for GIL removal or modification are in progress
-
Startup Time:
- Slower initialization compared to compiled languages
- Impacts microservice architectures and serverless functions
- Mitigated by persistent processes and optimized runtimes
-
Memory Usage:
- Higher baseline memory consumption
- Potential challenges in memory-constrained environments
- Optimization techniques and alternative implementations help address this
# Example of multiprocessing to overcome GIL limitations
import multiprocessing as mp
import numpy as np
def process_data_chunk(chunk):
# CPU-intensive processing
result = np.array([complex_calculation(x) for x in chunk])
return result.sum()
if __name__ == '__main__':
# Large dataset
data = np.random.random(10_000_000)
# Split into chunks for parallel processing
chunks = np.array_split(data, mp.cpu_count())
# Process in parallel
with mp.Pool() as pool:
results = pool.map(process_data_chunk, chunks)
# Combine results
final_result = sum(results)
Mobile and Client-Side Development
Python faces challenges in certain deployment environments:
-
Mobile Development:
- Limited native support on iOS and Android
- Frameworks like BeeWare and Kivy exist but with trade-offs
- Often used for backend services rather than mobile clients
-
Desktop Applications:
- Distribution complexity compared to web applications
- Performance considerations for graphics-intensive applications
- Packaging solutions have improved but challenges remain
-
Browser Execution:
- Pyodide and other WebAssembly solutions enable Python in browsers
- Performance overhead compared to JavaScript
- Primarily useful for specific use cases rather than general web development
Enterprise-Specific Challenges
Organizations adopting Python may encounter certain obstacles:
-
Legacy System Integration:
- Interfacing with older systems may require additional libraries
- Performance overhead in integration layers
- Documentation gaps for enterprise-specific technologies
-
Governance and Compliance:
- Supply chain security concerns with open-source packages
- Validation requirements in regulated industries
- Need for standardized development practices
-
Support and Maintenance:
- Dependency management in long-lived applications
- Version compatibility across large codebases
- Finding specialized expertise for complex implementations
Addressing Python's Limitations
The Python ecosystem has developed numerous strategies to address its challenges:
-
Performance Optimization:
- Just-In-Time compilation via PyPy and Numba
- Native extension modules for performance-critical code
- Vectorization and GPU acceleration libraries
-
Deployment Solutions:
- Containerization for consistent environments
- Compiled executable creators like PyInstaller and cx_Freeze
- Server-side rendering approaches for client applications
-
Enterprise Enablement:
- Commercial support offerings
- Enhanced security scanning tools
- Compliance documentation and certification paths
Python Community and Support
The Python Software Foundation
The Python Software Foundation (PSF) continues to play a vital role in Python's development and community:
-
Governance and Leadership:
- Transparent governance model with elected board
- Stewardship of the Python language and its evolution
- Fiscal sponsorship of key Python projects and initiatives
-
Community Support:
- Grants and sponsorships for community events
- Diversity and inclusion initiatives
- Educational outreach programs
-
Technical Infrastructure:
- Maintenance of python.org and PyPI
- Security response coordination
- Standards development and documentation
Conferences and Events
Python's vibrant conference ecosystem continues to thrive:
-
Global Events:
- PyCon: The flagship conference series with events worldwide
- EuroPython: Europe's principal Python gathering
- PyCon APAC: Asia-Pacific regional conference
- SciPy: Scientific computing focus
-
Specialized Conferences:
- PyData: Data science and analytics
- DjangoCon: Django framework community
- PyTorch Developer Conference: ML framework focus
- Python Web Conference: Web development concentration
-
Virtual and Hybrid Events:
- Online-first conferences with global participation
- Local meetups with virtual components
- Recorded content libraries from past events
Online Communities and Resources
Python's online presence provides abundant support options:
-
Discussion Forums and Q&A:
- Stack Overflow: Over 2 million Python questions answered
- Reddit communities: r/Python, r/learnpython, and specialized subreddits
- Discord and Slack channels for various Python communities
-
Documentation and Tutorials:
- Official Python documentation: Comprehensive and well-maintained
- Community tutorials and blogs
- Interactive learning platforms
-
Code Repositories and Sharing:
- GitHub: Python is consistently among the most popular languages
- Package repositories: PyPI, Conda-forge
- Code snippet sharing and collaboration tools
Open Source Contribution
Python's open-source nature remains a core strength:
-
Contribution Pathways:
- Core language development
- Library and framework maintenance
- Documentation and translation
- Mentoring and community support
-
Development Process:
- PEP (Python Enhancement Proposal) system for language changes
- Open discussion on Python mailing lists and GitHub
- Inclusive decision-making processes
-
Corporate Involvement:
- Major technology companies supporting Python development
- Funding for core developers and projects
- Infrastructure and resource donations
Future-Proofing Your Python Skills
Tracking Python's Evolution
Staying current with Python's development ensures long-term relevance:
-
Following Language Changes:
- PEP announcements and discussion
- Python developer surveys and roadmaps
- Release notes and deprecation warnings
-
Ecosystem Awareness:
- Package popularity and maintenance trends
- Emerging libraries and frameworks
- Integration with new technologies
-
Industry Application Evolution:
- Use case changes and new implementations
- Industry-specific Python adaptations
- Performance improvement approaches
Complementary Skills
Python professionals benefit from developing related skills:
-
Technical Complements:
- SQL and database design
- JavaScript for full-stack capabilities
- Docker and Kubernetes for deployment
- Git and version control mastery
-
Domain Knowledge:
- Business processes and requirements
- Industry-specific regulations and standards
- Data analytics and visualization principles
- Software engineering best practices
-
Soft Skills:
- Communication and documentation
- Collaboration and teamwork
- Problem-solving and critical thinking
- Project management and estimation
Continuous Learning Strategies
Effective approaches to ongoing Python development include:
-
Structured Learning:
- Online courses and certifications
- Books and technical publications
- Workshops and training sessions
-
Project-Based Learning:
- Personal projects exploring new concepts
- Open-source contributions
- Hackathons and coding challenges
-
Community Engagement:
- Meetup participation
- Conference attendance
- Forum discussion and question answering
- Mentoring and teaching others
Anticipating Future Trends
Several emerging trends will likely impact Python's future:
-
Language Evolution:
- Potential GIL removal or improvement
- Enhanced JIT compilation options
- Further type system refinements
- Improved concurrency models
-
Application Areas:
- Expanded use in edge computing
- Quantum computing algorithm development
- Advanced AI model creation and deployment
- Sustainability and green computing initiatives
-
Development Experience:
- AI-assisted coding and pair programming
- Advanced static analysis and security scanning
- Improved debugging and profiling tools
- Seamless cloud development environments
Conclusion
Why Python Remains Essential in 2025
Python's position as a crucial language to learn in 2025 is built on several enduring strengths:
-
Versatility and Applicability: Python spans domains from web development to data science, automation to artificial intelligence, making it a universally valuable skill regardless of your career direction.
-
Accessibility and Productivity: Its readable syntax and emphasis on developer experience translate to faster development cycles and lower barriers to entry, benefits that remain compelling in an increasingly complex technology landscape.
-
Ecosystem and Community: Python's vast library ecosystem and supportive community provide resources for nearly any task, ensuring solutions are readily available and knowledge is easily accessible.
-
Industry Adoption: From startups to enterprises, Python has established itself as a cornerstone technology in organizations of all sizes, creating abundant career opportunities across sectors.
-
Future Relevance: Python's continued evolution and central role in emerging technologies position it well for long-term relevance in the technology ecosystem.
Balanced Perspective
While Python offers numerous advantages, a realistic view acknowledges its limitations:
-
Performance trade-offs exist for certain use cases, though optimization options have improved dramatically.
-
Mobile and client-side development remain challenging, though server-side Python excels.
-
Specialized domains may benefit from purpose-built languages with different design priorities.
Despite these constraints, Python's strengths far outweigh its limitations for a vast array of applications, and its ecosystem continues to address these challenges through innovation and extension.
Taking the Next Step
For those convinced of Python's value, several paths forward exist:
-
For beginners: Start with the fundamentals through interactive tutorials and introductory courses, building a solid foundation before specializing.
-
For experienced developers: Leverage Python's approachability to quickly add it to your skillset, focusing on how it complements your existing expertise.
-
For organizations: Consider Python's potential to accelerate development cycles, unify disparate technical teams, and enable advanced data capabilities.
The journey with Python is inherently rewarding - not just for the practical skills acquired, but for joining a community that values readability, simplicity, and the joy of programming. In 2025 and beyond, Python remains a language that empowers individuals and organizations to solve problems efficiently, express ideas clearly, and build solutions that matter.
As the Zen of Python wisely states: "Simple is better than complex." In an increasingly complex technological world, Python's simplicity remains its most profound strength.
This article represents current trends and projections based on Python's trajectory through early 2025. Technology evolves rapidly, and readers are encouraged to supplement this information with the latest developments in the Python ecosystem.