Why Learning AI Programming is Worth It: Becoming a Pioneer in Artificial Intelligence
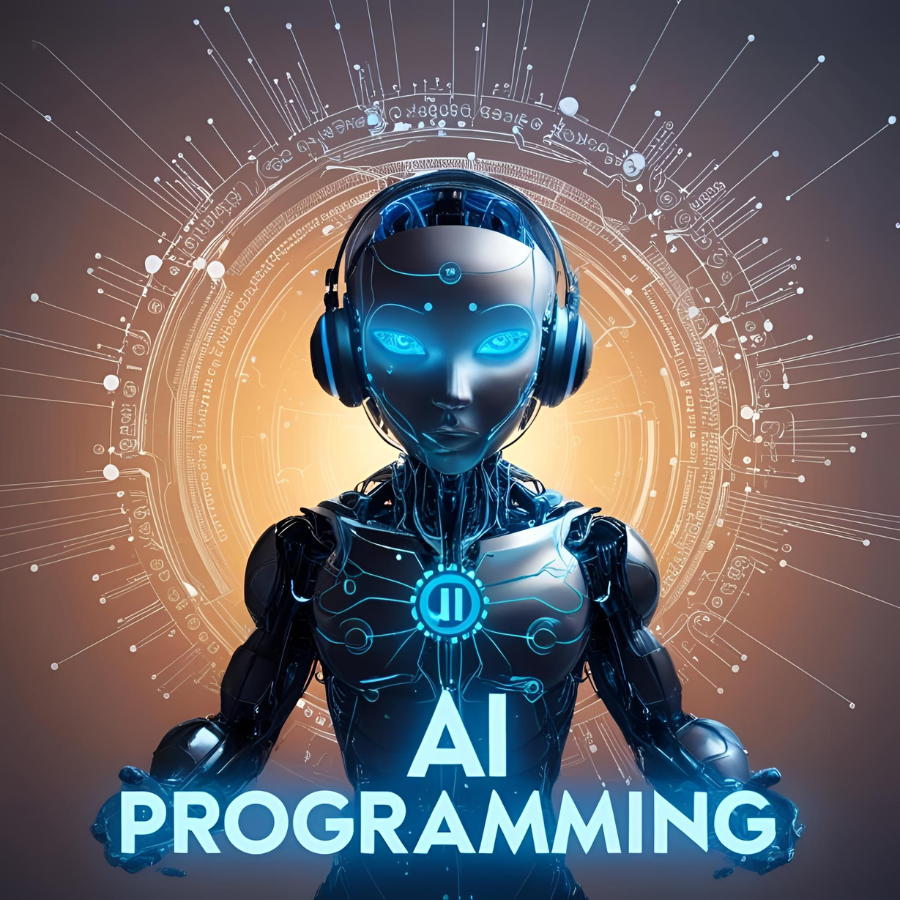
Introduction
In today's rapidly evolving technological landscape, artificial intelligence (AI) stands as the cornerstone of innovation, reshaping industries, economies, and societies at an unprecedented pace. The transformative power of AI extends beyond simple automation, venturing into territories once thought to be exclusively human domains—creativity, decision-making, pattern recognition, and complex problem-solving. As we witness this technological revolution unfold, one question emerges with increasing urgency: why should you learn AI programming, and what advantages come with being at the forefront of this field?
AI programming represents more than just another coding skill; it embodies the convergence of computer science, mathematics, cognitive psychology, and domain expertise. This interdisciplinary nature makes AI programming both intellectually stimulating and professionally valuable. By mastering AI programming, individuals position themselves at the intersection of innovation and practical application, capable of creating solutions that can fundamentally alter how we interact with technology and with each other.
The purpose of this comprehensive article is to explore the compelling reasons to learn AI programming and the significant benefits of becoming a pioneer in this dynamic field. We will delve into the current state of AI technology, the projected growth of the industry, the diverse career opportunities available, the intellectual challenges and rewards, and the potential for meaningful impact across various sectors. Whether you're a student contemplating your future path, a professional considering a career pivot, or simply a curious mind intrigued by the possibilities of AI, this article aims to provide you with a thorough understanding of why AI programming represents one of the most valuable skills in the 21st century.
The Current State and Future Trajectory of AI
The AI Revolution: Where We Stand Today
Artificial intelligence has transitioned from science fiction to an integral part of our daily lives. From voice assistants like Siri and Alexa to recommendation algorithms on streaming platforms, from autonomous vehicles to advanced diagnostic tools in healthcare, AI applications permeate virtually every aspect of modern society. The current state of AI reflects remarkable progress in areas such as:
- Machine Learning: Systems that learn from data and improve performance over time
- Natural Language Processing: Technologies that understand, interpret, and generate human language
- Computer Vision: Systems that can interpret and make decisions based on visual information
- Reinforcement Learning: Algorithms that learn optimal behaviors through trial and error
- Generative AI: Systems that can create new content, including text, images, music, and code
Major tech companies including Google, Microsoft, Amazon, and Meta have invested billions in AI research and development, recognizing its potential to drive future growth and innovation. Additionally, countless startups are emerging with AI-focused solutions across industries ranging from agriculture to finance, from education to entertainment.
Growth Projections and Market Trends
The economic impact of AI is projected to be transformative. According to research by PwC, AI could contribute up to $15.7 trillion to the global economy by 2030, with $6.6 trillion coming from increased productivity and $9.1 trillion from consumption-side effects. This represents a seismic shift in economic value creation, potentially increasing global GDP by up to 14%.
Several key trends are driving this growth:
- Enterprise AI Adoption: Companies across sectors are implementing AI solutions to improve efficiency, reduce costs, and enhance customer experiences.
- AI Democratization: The development of user-friendly AI platforms and tools is making the technology more accessible to non-specialists.
- Edge AI: The deployment of AI capabilities on local devices rather than in the cloud is enabling faster processing and greater privacy.
- AI Ethics and Governance: Increasing focus on developing responsible AI frameworks and regulations.
- Multimodal AI: Systems that can process multiple types of input (text, images, audio) are becoming more sophisticated.
The Widening AI Skills Gap
Despite this explosive growth, the industry faces a critical shortage of qualified AI professionals. According to a 2022 report by the World Economic Forum, AI specialist ranks among the top emerging jobs globally, with demand significantly outpacing supply. IBM's research indicates that the number of AI jobs will increase by 2.3 million by 2025, creating a substantial skills gap.
This skills shortage spans multiple domains:
- AI Researchers: Professionals advancing the theoretical foundations of AI
- Machine Learning Engineers: Specialists who build and deploy ML models
- Data Scientists: Experts who extract insights from data using AI techniques
- AI Product Managers: Professionals who guide the development of AI products
- AI Ethics Specialists: Experts focused on ensuring AI systems are fair, transparent, and accountable
For those entering the field now, this gap represents an extraordinary opportunity. Early movers in AI programming are positioned to benefit from high demand, competitive compensation, and the chance to shape the direction of this transformative technology.
Career Opportunities and Financial Rewards
The Expanding AI Job Market
The AI job market is not only growing rapidly but also diversifying across industries and roles. Key sectors actively recruiting AI talent include:
- Technology: Tech giants and startups developing core AI technologies and platforms
- Healthcare: Organizations implementing AI for diagnostics, drug discovery, and personalized medicine
- Finance: Institutions leveraging AI for fraud detection, algorithmic trading, and risk assessment
- Retail: Companies using AI for inventory management, customer analytics, and personalized shopping experiences
- Manufacturing: Businesses implementing AI for quality control, predictive maintenance, and supply chain optimization
- Transportation: Organizations developing autonomous vehicles and smart logistics solutions
- Education: Institutions creating adaptive learning platforms and automated assessment tools
- Entertainment: Companies using AI for content creation, recommendation systems, and immersive experiences
Within these sectors, numerous specialized roles have emerged:
- AI Research Scientist: Developing new algorithms and approaches to advance the field
- Machine Learning Engineer: Building and deploying ML models and systems
- AI Software Developer: Creating applications that incorporate AI capabilities
- Computer Vision Engineer: Specializing in systems that process and analyze visual information
- Natural Language Processing Specialist: Focusing on technologies that understand and generate human language
- Robotics Engineer: Designing AI-powered robotic systems
- AI Ethics Consultant: Ensuring AI systems are designed and deployed responsibly
- AI Product Manager: Guiding the development of AI-powered products and services
This diversity of roles means that individuals with different backgrounds and interests can find their niche within the AI ecosystem.
Salary Comparisons and Compensation Trends
AI professionals command some of the highest salaries in the technology sector. According to data from Glassdoor, LinkedIn, and industry reports, here's how AI-related positions compare to other tech roles:
Position | Average Annual Salary (USD) |
---|---|
AI Research Scientist | $120,000 - $200,000+ |
Machine Learning Engineer | $110,000 - $170,000 |
Data Scientist | $95,000 - $165,000 |
AI Software Developer | $105,000 - $160,000 |
Computer Vision Engineer | $115,000 - $180,000 |
NLP Specialist | $110,000 - $175,000 |
Traditional Software Engineer | $90,000 - $140,000 |
Web Developer | $70,000 - $120,000 |
These figures vary by location, experience level, and specific employer, but the premium for AI expertise is consistent across markets. Furthermore, compensation packages for AI specialists often include substantial equity, especially at startups, offering the potential for significant additional financial upside.
Long-term Career Growth and Stability
Beyond immediate financial rewards, AI programming offers exceptional long-term career prospects:
-
Recession Resistance: Even during economic downturns, companies typically continue to invest in technologies that provide competitive advantages or cost savings. AI falls squarely in this category.
-
Transferable Skills: The mathematical and computational thinking developed through AI programming transfers readily across industries and applications.
-
Ongoing Learning Opportunities: The field's rapid evolution ensures continuous intellectual challenges and growth opportunities.
-
Leadership Pathways: As AI becomes central to business strategy, AI specialists increasingly find themselves on accelerated paths to leadership roles, including Chief AI Officer, Chief Data Officer, and even CEO positions.
-
Entrepreneurial Potential: The combination of technical AI knowledge and domain expertise creates unique opportunities to identify and address unmet market needs through entrepreneurial ventures.
The Intellectual Challenge and Fulfillment
The Interdisciplinary Nature of AI
One of the most intellectually stimulating aspects of AI programming is its deeply interdisciplinary character. Mastering AI requires engagement with:
- Computer Science: Understanding algorithms, data structures, and computational complexity
- Mathematics: Applying linear algebra, calculus, probability, and statistics
- Cognitive Science: Incorporating insights about human learning and intelligence
- Domain Expertise: Developing deep knowledge of the specific field where AI is being applied
- Ethics and Philosophy: Grappling with questions about fairness, accountability, and the impact of AI systems
This interdisciplinary approach means that AI programming never becomes routine or monotonous. Each project presents unique challenges that engage different aspects of your knowledge and creativity.
The Problem-Solving Mindset
AI programming cultivates a particular problem-solving mindset that combines analytical rigor with creative thinking. This approach involves:
- Problem Framing: Defining the problem in a way that makes it amenable to AI solutions
- Data Understanding: Developing deep insights into the data that will train your models
- Model Selection and Design: Choosing appropriate algorithms and architectures for the specific problem
- Experimental Thinking: Designing experiments to test hypotheses about model performance
- Iterative Improvement: Continuously refining approaches based on empirical results
- Systems Thinking: Understanding how AI components interact with broader technical and social systems
This comprehensive problem-solving framework extends beyond AI programming itself, becoming a valuable approach to challenges in many domains of life and work.
The Thrill of Innovation and Discovery
Few fields offer the opportunity for genuine innovation and discovery that AI programming provides. As an AI programmer, you might:
- Develop a novel algorithm that improves performance on a challenging task
- Discover unexpected patterns in complex datasets
- Create a new application of AI that transforms an industry
- Contribute to fundamental research that advances our understanding of intelligence
This potential for groundbreaking work creates a sense of excitement and fulfillment that distinguishes AI programming from many other professional pursuits. The field's rapid pace of advancement means that even relatively junior practitioners can make meaningful contributions to the state of the art.
Impact and Contribution Opportunities
Addressing Global Challenges with AI
AI programming offers the opportunity to contribute to solutions for some of humanity's most pressing challenges:
- Climate Change: AI systems for optimizing energy use, designing efficient materials, modeling climate patterns, and monitoring environmental changes
- Healthcare: AI applications for early disease detection, personalized treatment plans, drug discovery, and healthcare resource allocation
- Food Security: AI-powered agricultural solutions for crop monitoring, precision farming, supply chain optimization, and reducing food waste
- Education: Adaptive learning systems that personalize education to individual needs and make quality instruction more accessible
- Economic Inequality: AI tools that expand economic opportunities, improve financial inclusion, and enhance workforce development
These applications demonstrate how AI programming skills can be directed toward creating social good and addressing complex global problems.
Industry Transformation and Innovation
Beyond addressing global challenges, AI programming enables you to participate in the transformation of industries and the creation of entirely new business models:
- Healthcare: Revolutionizing diagnosis, treatment planning, and drug discovery
- Transportation: Enabling autonomous vehicles and optimizing logistics networks
- Finance: Transforming risk assessment, fraud detection, and investment strategies
- Retail: Reinventing customer experiences through personalization and automation
- Manufacturing: Enhancing efficiency through predictive maintenance and quality control
- Creative Industries: Enabling new forms of content creation and audience engagement
By learning AI programming, you position yourself to be part of these transformative changes, helping to shape how industries evolve rather than merely reacting to changes after they occur.
Personal Projects and Entrepreneurial Ventures
AI programming skills empower you to pursue personal projects and entrepreneurial ventures with significant potential impact:
- Developing applications that solve specific problems in your community or industry
- Creating open-source tools that advance the accessibility and capability of AI technologies
- Founding startups focused on novel AI applications for underserved markets
- Consulting with organizations to help them implement AI solutions effectively
- Teaching and mentoring to help others develop AI skills and ethical frameworks
These possibilities highlight how AI programming enables not just participation in existing initiatives but the creation of entirely new paths for impact and contribution.
The First-Mover Advantage in AI
Establishing Yourself as an AI Expert
Being among the first to develop expertise in AI programming offers substantial advantages in establishing yourself as a recognized expert:
- Thought Leadership Opportunities: Early specialists have unparalleled opportunities to publish, speak at conferences, and shape discourse in the field
- Professional Network Development: Pioneering AI programmers typically develop extensive networks with other innovators and decision-makers
- Personal Brand Building: First movers can build personal brands associated with specific AI applications or approaches
- Visibility to Key Stakeholders: Early expertise attracts attention from investors, industry leaders, and research institutions
- Reputation for Innovation: Being known as someone who adopts and masters emerging technologies creates a valuable professional reputation
These advantages compound over time, as early expertise often leads to opportunities that further enhance your knowledge, network, and reputation.
Setting Industry Standards and Practices
First movers in AI programming frequently have the opportunity to influence how the field develops:
- Technical Standards: Contributing to frameworks, libraries, and protocols that become industry standards
- Best Practices: Establishing approaches to data management, model development, and system evaluation
- Ethical Guidelines: Helping to define responsible AI development and deployment
- Educational Pathways: Shaping how AI programming is taught and learned
- Regulatory Frameworks: Informing policymakers about technical realities that should guide regulation
This influence extends far beyond personal career advantages, allowing you to shape the direction of the field in ways that align with your values and vision.
Capturing Emerging Market Opportunities
Early expertise in AI programming positions you to identify and pursue emerging market opportunities before they become obvious to the mainstream:
- Spotting Unaddressed Needs: Recognizing problems that AI can solve before others see the opportunity
- Creating Novel Applications: Developing innovative uses of AI in domains where it hasn't yet been applied
- Forming Strategic Partnerships: Establishing relationships with key players in emerging AI ecosystems
- Securing Early Funding: Attracting investment for AI ventures before the space becomes crowded
- Building Competitive Moats: Developing proprietary data, algorithms, or domain knowledge that creates sustainable advantages
These market opportunities often represent the intersection of specific domain knowledge and AI capabilities—a sweet spot for pioneers who combine technical expertise with industry insights.
Learning Pathways and Resources
Structured Educational Options
Multiple structured educational pathways can help you develop AI programming expertise:
-
University Degrees:
- Bachelor's degrees in Computer Science, Data Science, or AI (emerging)
- Master's programs in Machine Learning, AI, or related fields
- PhD programs for those pursuing research careers
-
Professional Certificates:
- Google's Machine Learning Engineer certification
- Microsoft's Azure AI Engineer Associate
- IBM's AI Engineering Professional Certificate
- NVIDIA's Deep Learning Institute certifications
- AWS Machine Learning Specialty certification
-
Bootcamps and Intensive Programs:
- AI-focused coding bootcamps (12-24 weeks)
- University extension programs
- Industry-sponsored training programs
Each of these options offers different advantages in terms of depth, recognition, networking opportunities, and time investment.
Self-Directed Learning Resources
For self-directed learners, numerous high-quality resources are available:
-
Online Courses:
- Andrew Ng's Machine Learning courses on Coursera
- Deep Learning Specialization from deeplearning.ai
- Fast.ai's practical deep learning courses
- MIT and Stanford's open courseware on AI topics
- Udacity's AI Programming with Python Nanodegree
-
Books:
- "Hands-On Machine Learning with Scikit-Learn, Keras, and TensorFlow" by Aurélien Géron
- "Deep Learning" by Ian Goodfellow, Yoshua Bengio, and Aaron Courville
- "Artificial Intelligence: A Modern Approach" by Stuart Russell and Peter Norvig
- "Python Machine Learning" by Sebastian Raschka
- "The Hundred-Page Machine Learning Book" by Andriy Burkov
-
Online Communities and Forums:
- Kaggle (competitions and tutorials)
- GitHub (open-source projects)
- Stack Overflow (problem-solving)
- Reddit communities (r/MachineLearning, r/datascience)
- AI research paper discussion groups
-
Hands-on Practice Platforms:
- Google Colab (free GPU access)
- Kaggle Kernels
- Hugging Face Spaces
- Paperspace Gradient
- Local development environments
Practical Project Development
Regardless of your learning pathway, developing practical projects is essential for mastering AI programming:
-
Starter Projects:
- Image classification with basic datasets (MNIST, CIFAR-10)
- Sentiment analysis on review datasets
- Simple time series forecasting
- Recommendation systems with movie or product data
- Game-playing agents for simple games
-
Intermediate Projects:
- Natural language processing for text summarization or question answering
- Computer vision applications (object detection, image segmentation)
- Reinforcement learning for complex control tasks
- Generative models for creating images or text
- Anomaly detection systems
-
Advanced Projects:
- Multi-modal AI systems combining text, image, and audio
- Transfer learning approaches for low-data domains
- Custom model architectures for specific applications
- End-to-end AI products with user interfaces
- Domain-specific AI solutions (healthcare, finance, etc.)
Building a portfolio of increasingly sophisticated projects demonstrates your skills to potential employers or clients while deepening your practical understanding of AI programming concepts.
Overcoming Common Concerns and Challenges
The Learning Curve and Prerequisites
Many prospective AI programmers worry about the steepness of the learning curve. While AI programming does require substantial knowledge, the path can be broken down into manageable steps:
-
Mathematical Foundations:
- Linear algebra (vectors, matrices, operations)
- Calculus (derivatives, gradients, optimization)
- Probability and statistics (distributions, inference, hypothesis testing)
-
Programming Fundamentals:
- Python programming (the dominant language in AI)
- Data structures and algorithms
- Software engineering principles
-
Machine Learning Basics:
- Supervised and unsupervised learning
- Model evaluation and validation
- Feature engineering
-
Deep Learning and Advanced Topics:
- Neural network architectures
- Training and optimization techniques
- Specialized approaches (NLP, computer vision, reinforcement learning)
Rather than attempting to master everything at once, focus on building a strong foundation and then expanding your knowledge incrementally through practical applications.
Time Investment and Resource Requirements
Developing AI programming expertise requires a significant time investment, but several strategies can make this more manageable:
- Consistent Practice: Even 30-60 minutes daily can yield substantial progress over time
- Project-Based Learning: Focus on completing specific projects rather than abstract study
- Community Engagement: Learning with others accelerates progress and maintains motivation
- Leveraging Existing Code: Building on open-source projects rather than starting from scratch
- Cloud Resources: Using cloud platforms for computation rather than investing in expensive hardware
For those concerned about hardware requirements, options like Google Colab, Kaggle Kernels, and AWS SageMaker provide access to powerful computing resources at low or no cost for learning purposes.
Navigating the Rapidly Evolving Landscape
The rapid evolution of AI can make it challenging to know what to focus on and how to stay current:
- Focus on Fundamentals: Core principles of machine learning and AI remain relatively stable even as specific techniques evolve
- Develop a Learning System: Set aside regular time to review new developments through research papers, blogs, and courses
- Follow Key Researchers and Organizations: Identify influential figures and organizations in your areas of interest
- Participate in Community Events: Attend conferences, workshops, and meetups (virtually or in person)
- Contribute to Open Source: Engaging with open-source projects provides visibility into cutting-edge developments
Remember that no one can keep up with everything in AI—even leading researchers specialize in particular subfields. Developing depth in specific areas while maintaining awareness of broader trends is a sustainable approach.
The Ethical Dimension of AI Leadership
Responsible AI Development
As an early mover in AI programming, you have the opportunity and responsibility to champion ethical AI development:
- Fairness and Bias Mitigation: Ensuring AI systems don't perpetuate or amplify existing social biases
- Transparency and Explainability: Creating systems whose decisions can be understood and scrutinized
- Privacy Protection: Designing AI that respects privacy and gives users control over their data
- Safety and Robustness: Building systems that behave reliably even in unexpected situations
- Human-Centered Design: Developing AI that augments human capabilities rather than simply replacing humans
These principles are not merely ethical considerations but increasingly critical business factors as users, customers, and regulators demand more responsible AI.
Shaping the Future of AI Governance
First movers in AI programming have unique opportunities to influence how AI is governed:
- Participating in Standard-Setting Bodies: Contributing to IEEE, ISO, and other standards organizations
- Engaging with Policy Discussions: Providing technical expertise to policymakers
- Developing Industry Best Practices: Creating and promoting responsible approaches within your organization
- Contributing to Open Governance Initiatives: Supporting transparency in AI development
- Educating Stakeholders: Helping non-technical decision-makers understand AI capabilities and limitations
This engagement ensures that AI governance is informed by technical reality while remaining aligned with broader societal values.
Creating Inclusive AI Communities
As a pioneer in AI programming, you can help build more inclusive communities that bring diverse perspectives to AI development:
- Mentoring Underrepresented Groups: Supporting individuals from diverse backgrounds
- Making Learning Resources Accessible: Creating or promoting resources that reduce barriers to entry
- Advocating for Inclusive Teams: Championing diversity in hiring and promotion
- Addressing Cultural Context: Ensuring AI systems work well across different cultural settings
- Designing for Accessibility: Creating AI systems usable by people with disabilities
This commitment to inclusion isn't just about fairness—it directly enhances the quality and applicability of AI systems by incorporating broader perspectives and use cases.
Case Studies: Success Stories in AI Pioneering
Individual Transformation Stories
The transformative potential of AI programming is illustrated by numerous individual success stories:
Case 1: From Traditional Software to AI Innovation
Sarah K., Software Engineer
Sarah had worked as a traditional software developer for five years when she decided to invest in learning machine learning and deep learning. Within 18 months of focused study and project work, she transitioned to a role as a machine learning engineer at a leading tech company, increasing her compensation by 40% and finding significantly more engaging work.
Case 2: Domain Expertise Enhanced by AI
Dr. Michael T., Healthcare Professional
As a practicing radiologist, Dr. Michael recognized the potential of AI in medical imaging. He spent evenings and weekends learning AI programming, focusing specifically on computer vision applications. Within two years, he co-founded a startup applying AI to early cancer detection, which was later acquired for $30 million.
Case 3: Career Transition from Unrelated Field
Ricardo J., Former Marketing Analyst
Ricardo had no technical background when he became fascinated by natural language processing. Starting with basic Python, he gradually built expertise in NLP through online courses and personal projects. Three years later, he leads an AI team developing sentiment analysis tools for a major market research firm.
Organizational Innovation Through AI Leadership
Organizations led by AI pioneers have achieved remarkable transformations:
Case 1: Manufacturing Efficiency Revolution
A traditional manufacturing company facing intense competition invested in AI expertise, hiring several pioneering AI programmers. These early adopters developed predictive maintenance systems that reduced downtime by 37% and custom quality control systems that decreased defect rates by 42%, fundamentally changing the company's competitive position.
Case 2: Healthcare Diagnostic Breakthroughs
A medical technology startup led by AI programming pioneers developed diagnostic algorithms that could detect certain conditions from medical images with greater accuracy than experienced specialists. Their approach has been integrated into diagnostic workflows at over 200 hospitals, improving patient outcomes while reducing costs.
Case 3: Financial Services Transformation
A regional bank that prioritized AI programming expertise early was able to develop sophisticated fraud detection systems and personalized financial advice platforms years before competitors. This early adoption contributed to a 22% increase in customer retention and a 28% reduction in fraud losses.
Startup Success Through AI Innovation
The startup landscape is filled with examples of successful ventures founded by AI programming pioneers:
Case 1: Natural Language Processing Innovation
Founded by early specialists in NLP, this startup developed an AI writing assistant that helps people create more effective business communications. Within four years, they reached 5 million users and a $500 million valuation.
Case 2: Computer Vision Application
Two computer vision researchers created a startup applying their expertise to retail inventory management. Their system, which automatically tracks stock levels using ordinary security cameras, was adopted by major retail chains and led to an acquisition valued at over $100 million.
Case 3: AI for Sustainable Agriculture
A team with combined expertise in machine learning and agronomy developed AI systems for precision farming that reduce water usage by up to 30% while increasing crop yields. Their company has grown to serve customers across three continents and raised $75 million in venture funding.
These case studies demonstrate that pioneering AI programming expertise leads to opportunities ranging from individual career advancement to organizational transformation to entrepreneurial success.
Conclusion: The Path Forward
Synthesizing the Value Proposition
Learning AI programming and positioning yourself as a pioneer in the field offers a compelling value proposition that spans multiple dimensions:
- Economic Opportunity: Access to high-demand, well-compensated roles across industries
- Intellectual Fulfillment: Engagement with complex, interdisciplinary challenges at the frontier of technology
- Impact Potential: Ability to contribute to solutions for significant global and local problems
- Career Resilience: Development of skills likely to remain valuable through technological and economic changes
- Influence and Leadership: Opportunity to shape the direction of a transformative technology
These benefits are amplified for early movers who develop expertise ahead of mainstream adoption, providing advantages in professional reputation, network development, and access to emerging opportunities.
Taking the First Steps
The journey to AI programming expertise begins with concrete first steps:
- Assess Your Starting Point: Evaluate your existing knowledge in mathematics, programming, and relevant domains
- Set Clear Learning Goals: Define specific skills you want to develop and projects you want to complete
- Create a Structured Plan: Develop a learning roadmap with milestones and timelines
- Build a Support System: Connect with communities, mentors, or peers on similar journeys
- Start Small but Start Now: Begin with manageable projects that build confidence and momentum
Remember that becoming an AI pioneer doesn't require instant mastery of all aspects of the field. Many successful AI professionals began with focused expertise in specific applications or techniques, expanding their knowledge over time.
The Ongoing Journey of Learning and Innovation
Perhaps the most exciting aspect of AI programming is that it represents not a destination but an ongoing journey of learning and innovation. The field continues to evolve rapidly, with new techniques, applications, and challenges emerging regularly. This dynamic nature means that AI programming never becomes routine or stagnant—there are always new frontiers to explore, new problems to solve, and new capabilities to develop.
By choosing to learn AI programming and position yourself as a pioneer in the field, you're not just acquiring a valuable skill set for today's market. You're embarking on an intellectual adventure that can span your entire career, offering continuous opportunities for growth, impact, and fulfillment. In a world increasingly shaped by artificial intelligence, those who understand and can create these technologies will have unparalleled opportunities to influence how we live, work, and solve our most pressing challenges.
The question is not whether AI will transform our world—that transformation is already underway. The question is whether you will be among those shaping that transformation or merely experiencing its effects. By developing AI programming expertise now, you position yourself to be a creator of the future rather than simply an inhabitant of it.