Why Learning Python Programming Is Still Worth It in 2025: A Comprehensive Guide to a Lucrative Career Path
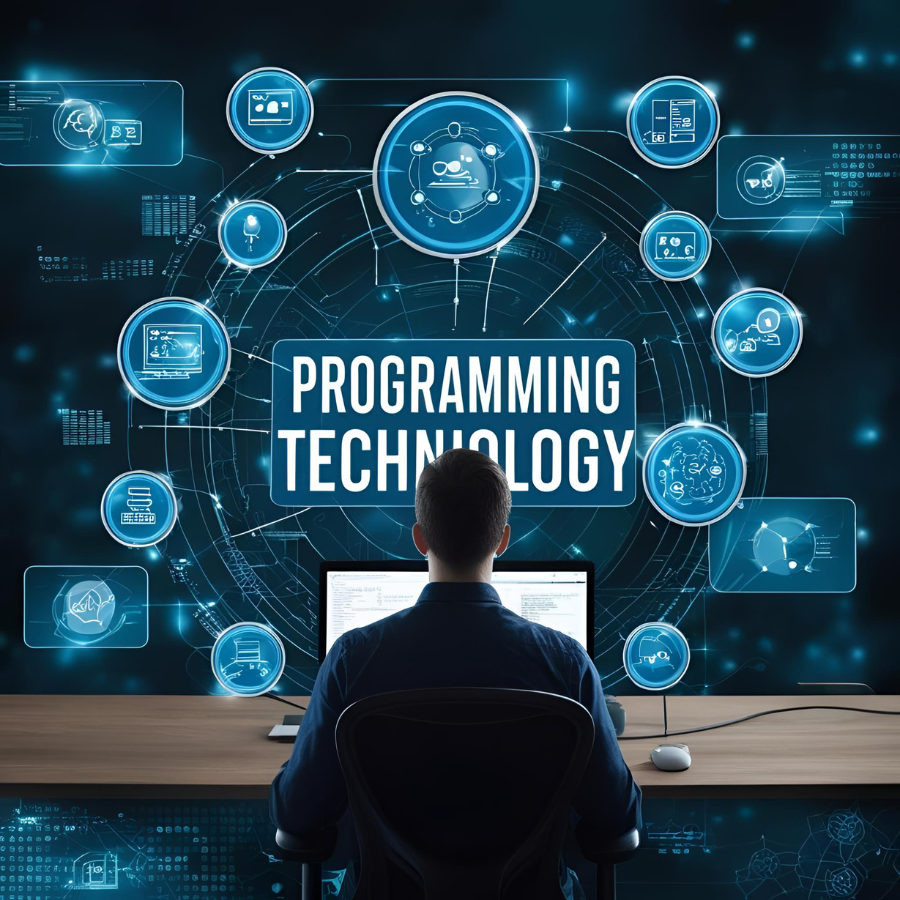
Introduction
In the ever-evolving landscape of technology, programming languages rise and fall in popularity, but Python has maintained its position as one of the most versatile and sought-after languages in the industry. As we approach 2025, many aspiring developers and professionals looking to switch careers might wonder: "Is Python still worth learning?" The short answer is a resounding yes. This comprehensive guide explores why Python continues to be a valuable skill, how it contributes to a lucrative career path, and why its dominance is expected to continue well into the future.
Python's journey from a simple scripting language to one of the pillars of modern software development is nothing short of remarkable. Created by Guido van Rossum in the late 1980s and named after the British comedy group Monty Python, this language has transcended its humble beginnings to become the backbone of many technological innovations that shape our world today. From web development to artificial intelligence, from data science to automation, Python's fingerprints are everywhere in the technology ecosystem.
In this article, we'll dive deep into the reasons why Python skills will remain highly valuable in 2025 and beyond. We'll explore the growing job market for Python developers, the impressive salary prospects, the language's versatility across different industries, and its continued evolution to meet modern technological demands. Whether you're a complete beginner contemplating your first programming language or an experienced developer considering adding Python to your toolkit, this guide will provide you with comprehensive insights to make an informed decision about your career path.
Python's Enduring Popularity: Market Trends and Statistics
Current Market Position
As of 2024, Python consistently ranks among the top three programming languages globally. According to the TIOBE Index, which measures language popularity based on search engine queries, Python has maintained its position in the top three for several consecutive years, often competing directly with languages like Java and C for the top spot. Similarly, the IEEE Spectrum ranking, which analyzes multiple metrics including job listings and open-source repositories, has repeatedly placed Python at or near the top of its list of programming languages.
The GitHub Octoverse report, which provides insights into the world's largest collection of open-source software, has shown consistent growth in Python repositories year over year. Stack Overflow's annual Developer Survey has also highlighted Python as one of the most loved and wanted programming languages, with developers expressing both satisfaction with using it and a desire to learn it.
Growth Projections for 2025 and Beyond
Looking ahead to 2025, several indicators suggest that Python's popularity will continue to grow:
- The U.S. Bureau of Labor Statistics projects a 22% growth in software developer jobs between 2020 and 2030, significantly faster than the average for all occupations. Python developers are poised to benefit from this expansion.
- Global market research firms predict that the artificial intelligence market will grow at a compound annual growth rate (CAGR) of around 40% through 2025, with Python being the dominant language in AI and machine learning development.
- The data science field, where Python reigns supreme, is expected to see continued expansion, with a projected market size of over $230 billion by 2026, according to various market research reports.
- Cloud computing adoption continues to accelerate, with Python being one of the primary languages for developing cloud applications and infrastructure as code.
Python in Comparison to Other Languages
When compared to other popular programming languages, Python demonstrates several advantages that contribute to its sustained growth:
- Unlike languages such as Java or C++, which have steeper learning curves, Python's readability and simplicity make it accessible to beginners while still offering powerful capabilities for experts.
- Compared to JavaScript, which dominates front-end web development, Python offers broader applicability across different domains, from web backends to scientific computing.
- Languages like R, which compete with Python in data analysis, don't offer the same versatility for general-purpose programming.
- Newer languages like Rust or Go, while excellent for specific use cases like systems programming or concurrent applications, haven't achieved Python's widespread adoption across multiple domains.
The combination of Python's established presence and its continued growth in emerging fields suggests that its dominance will extend well into 2025 and beyond, making it a safe and valuable language to learn for career prospects.
High Demand, High Rewards: Python Job Market Analysis
Current Job Market Landscape
The job market for Python developers remains robust and continues to expand. According to major job posting sites like Indeed, LinkedIn, and Glassdoor, Python consistently appears among the most requested skills in technology job listings. This demand spans various roles beyond just "Python Developer," including:
- Data Scientists and Data Analysts
- Machine Learning Engineers
- DevOps and Site Reliability Engineers
- Full-Stack Developers
- Automation Engineers
- Research Scientists
- Quality Assurance Engineers
- Financial Analysts and Quantitative Researchers
This diversity of roles highlights Python's versatility and explains why proficiency in this language opens doors to multiple career paths. Furthermore, Python developers often enjoy the advantage of remote work opportunities, as the language's popularity is global rather than confined to specific tech hubs.
Salary Prospects for Python Developers in 2025
Python developers continue to command impressive salaries, and this trend is expected to strengthen by 2025. Based on current trajectories and adjusting for inflation:
- Entry-level Python developers in the United States can expect salaries starting at approximately $80,000-$95,000 per year by 2025.
- Mid-level Python developers with 3-5 years of experience may earn between $110,000 and $140,000 annually.
- Senior Python developers and specialists in high-demand fields like machine learning or cloud architecture could command salaries exceeding $160,000 to $200,000+.
- Contract and freelance Python developers often charge between $70 and $150 per hour, depending on specialization and experience.
Globally, while absolute figures vary by region, Python developers typically earn above-average salaries for their local markets. In European tech hubs like London, Berlin, and Amsterdam, Python developer salaries are projected to range from €55,000 for juniors to €120,000+ for seniors by 2025. In growing tech markets like India, salaries for Python specialists are expected to increase at rates exceeding general software development roles.
Industry Demand Analysis
Python's demand spans various industries, each valuing different aspects of the language:
-
Finance and Fintech: Financial institutions increasingly rely on Python for algorithmic trading, risk analysis, and building fintech applications. By 2025, the integration of machine learning in financial services will further boost demand for Python skills in this sector.
-
Healthcare and Biotech: Python's role in processing medical data, genomics research, and healthcare analytics continues to grow. The pandemic accelerated digitalization in healthcare, creating sustained demand for Python developers.
-
E-commerce and Retail: Companies use Python for backend systems, recommendation engines, and supply chain optimization. As personalization becomes more sophisticated, Python developers who can work with large datasets and create predictive models will be in high demand.
-
Entertainment and Media: Content recommendation algorithms, media processing tools, and streaming platforms often utilize Python. The growth of interactive and personalized media will create more opportunities in this sector.
-
Manufacturing and Industry 4.0: Python's role in industrial automation, IoT applications, and predictive maintenance is expanding, creating new job categories that didn't exist a decade ago.
The breadth of industries seeking Python talent creates a safety net for developers - if one sector experiences a downturn, opportunities remain abundant in others, making Python a particularly resilient career choice heading into 2025.
Python's Versatility: Why It Remains the Swiss Army Knife of Programming
Cross-Domain Applications
One of Python's greatest strengths is its applicability across numerous domains, making it a uniquely versatile language that continues to find new applications. This adaptability is expected to increase its value further by 2025:
Web Development
While JavaScript dominates frontend development, Python remains a powerful choice for backend web development. Frameworks like Django and Flask continue to evolve and maintain strong communities. By 2025, these frameworks will have further matured, offering even more robust solutions for building web applications:
- Django's "batteries-included" approach provides a comprehensive framework that accelerates development while maintaining security best practices.
- Flask's lightweight and modular design allows developers to build precisely what they need without unnecessary overhead.
- FastAPI has gained significant traction for creating high-performance APIs with automatic interactive documentation.
The continued growth of web applications as business solutions ensures Python's relevance in this domain for years to come.
Data Science and Analytics
Python has established itself as the de facto language for data science, a position that will only strengthen by 2025:
- Libraries like Pandas, NumPy, and SciPy provide robust tools for data manipulation and scientific computing.
- Visualization libraries such as Matplotlib, Seaborn, and Plotly enable data scientists to create compelling visual representations of complex datasets.
- The integration of Python with big data technologies like Spark allows for processing massive datasets that would be unmanageable with traditional methods.
As organizations increasingly base decisions on data analysis, Python's role in transforming raw information into actionable insights becomes more critical.
Machine Learning and Artificial Intelligence
Python leads the AI revolution, with frameworks that make implementing complex algorithms accessible:
- TensorFlow, PyTorch, and Scikit-learn continue to expand their capabilities while maintaining Python as their primary interface.
- Natural Language Processing (NLP) applications, powered by libraries like NLTK, spaCy, and transformers, are revolutionizing how machines understand human language.
- Computer vision applications built with OpenCV and deep learning frameworks enable everything from facial recognition to medical image analysis.
By 2025, as AI becomes more embedded in everyday applications, Python's position as the gateway to implementing these technologies will further cement its value.
Automation and Scripting
Python excels at automating repetitive tasks, a capability increasingly valuable as organizations seek efficiency:
- System administrators use Python to automate configuration management and monitoring.
- DevOps engineers employ Python for continuous integration/continuous deployment (CI/CD) pipelines.
- Business analysts leverage Python to automate report generation and data collection.
This aspect of Python becomes increasingly valuable as automation extends beyond traditional IT roles into various business functions.
Ecosystem Advantages
Python's rich ecosystem of libraries and frameworks is a major factor in its continued relevance:
Comprehensive Standard Library
Python's "batteries included" philosophy means developers can accomplish many tasks without installing additional packages. The standard library includes modules for file I/O, web servers, data serialization, and more, reducing dependency management overhead.
Vast Third-Party Library Collection
With over 350,000 packages available through the Python Package Index (PyPI), developers rarely need to build solutions from scratch. This vast collection continues to grow, with new libraries emerging to address evolving needs:
- Scientific computing: Beyond the established NumPy and SciPy, specialized libraries for fields like astronomy, genetics, and economics continue to emerge.
- Web development: New frameworks and tools appear regularly, addressing specific needs like real-time applications or improved performance.
- Developer productivity: Tools for testing, documentation, and deployment constantly evolve to improve the development experience.
Framework Maturity and Evolution
By 2025, Python's major frameworks will have undergone further refinement, addressing previous limitations:
- Django will continue enhancing its async capabilities, improving performance for applications requiring high concurrency.
- Data science libraries will offer better integration with JavaScript visualization libraries for web-based data applications.
- Machine learning frameworks will provide more accessible interfaces for non-specialists while maintaining advanced capabilities for experts.
This combination of versatility across domains and a rich, evolving ecosystem ensures Python will remain relevant and valuable well beyond 2025.
Python in Emerging Technologies: Shaping the Future
Artificial Intelligence and Machine Learning Advancements
Python's dominance in AI and ML is projected to strengthen as these fields continue to evolve. By 2025, several developments will further enhance Python's position:
AI Democratization
As artificial intelligence becomes more accessible to non-specialists, Python's readability and relatively flat learning curve position it as the gateway language for AI implementation. Python libraries are increasingly offering higher-level abstractions that allow developers to implement sophisticated AI capabilities with minimal code:
- AutoML frameworks in Python are evolving to automate the process of algorithm selection and hyperparameter tuning.
- Pre-trained models available through Python interfaces enable developers to implement complex capabilities like computer vision or natural language understanding without extensive ML expertise.
- Transfer learning techniques, primarily implemented in Python, allow organizations to leverage existing models and adapt them to specific domains with limited data.
Specialized AI Applications
Python is at the forefront of specialized AI applications that are expected to see significant growth by 2025:
- Reinforcement learning implementations in Python are powering advances in robotics, game playing, and optimization problems.
- Generative AI models, built primarily with Python frameworks like PyTorch and TensorFlow, are revolutionizing content creation across text, images, audio, and video.
- Explainable AI tools, often built in Python, address the growing need for transparency and interpretability in AI systems for regulatory compliance and ethical considerations.
Edge AI Development
As AI moves from centralized servers to edge devices, Python is adapting to meet these new requirements:
- TensorFlow Lite and similar Python-based frameworks enable developers to deploy models on resource-constrained devices.
- Python tools for model optimization and quantization allow AI capabilities to run efficiently on smartphones, IoT devices, and embedded systems.
- Hybrid cloud-edge AI architectures, often orchestrated using Python, enable sophisticated applications that balance local processing with cloud capabilities.
Data Science Evolution
The data science field continues to expand in scope and importance, with Python as its primary language:
BigData Integration
Python's integration with big data technologies is strengthening, addressing previous limitations:
- PySpark improvements offer near-native performance when working with massive datasets.
- Dask and similar libraries provide Python-native solutions for parallel and distributed computing.
- Integration with cloud-based data warehouses allows Python code to efficiently query petabyte-scale datasets.
Automated Data Science
Python is leading the automation of the data science workflow:
- Automated feature engineering libraries reduce the manual effort in preparing data for analysis.
- Automated model selection and hyperparameter optimization make sophisticated modeling more accessible.
- Data validation and quality assurance libraries help ensure reliable inputs to data pipelines.
Real-time Analytics
Python's capabilities for real-time data processing are expanding:
- Integration with streaming platforms like Kafka enables Python applications to process data as it's generated.
- Libraries for online learning allow models to adapt continuously as new data arrives.
- Real-time visualization tools built in Python help organizations monitor key metrics and respond quickly to changes.
IoT and Edge Computing
Python's role in the Internet of Things (IoT) ecosystem is growing, challenging the traditional dominance of lower-level languages in this space:
MicroPython and CircuitPython
These Python variants designed for microcontrollers are gaining traction:
- MicroPython and CircuitPython support an increasing range of hardware platforms.
- The simplicity of Python reduces development time for IoT applications compared to C or C++.
- Libraries for common sensors and actuators simplify interaction with physical hardware.
Edge Data Processing
Python is increasingly used for processing data at the edge:
- Lightweight machine learning models in Python can run directly on edge devices.
- Python scripts can preprocess and filter data before transmission to reduce bandwidth requirements.
- Edge analytics applications written in Python enable real-time decision making without cloud dependency.
IoT Platform Integration
Major IoT platforms now offer Python SDKs and integration:
- Cloud IoT platforms from Amazon, Google, and Microsoft provide comprehensive Python support.
- Python libraries simplify device provisioning, management, and security.
- Python-based tools enable simulation and testing of IoT systems before deployment.
Quantum Computing
As quantum computing moves from theoretical to practical applications, Python is emerging as the preferred high-level language for this domain:
Quantum Development Frameworks
Major quantum computing frameworks prioritize Python interfaces:
- Qiskit (IBM), Cirq (Google), and Pennylane offer Python APIs for quantum algorithm development.
- Python's clear syntax makes quantum concepts more accessible to developers new to the field.
- Classical simulation of quantum circuits, a crucial development step, is primarily done in Python.
Quantum Machine Learning
The intersection of quantum computing and machine learning is an emerging field where Python plays a central role:
- Quantum machine learning libraries in Python enable researchers to explore quantum advantages in AI.
- Hybrid classical-quantum algorithms, a practical approach given current quantum hardware limitations, are typically implemented in Python.
- Tools for comparing classical and quantum approaches to the same problem are built using Python's scientific ecosystem.
Quantum Education and Research
Python facilitates learning and experimentation in quantum computing:
- Educational resources for quantum computing predominantly use Python for examples and exercises.
- Research papers in quantum computing often include Python code to demonstrate concepts.
- Quantum computing simulators in Python allow exploration of quantum algorithms without access to quantum hardware.
These emerging technologies represent fields with significant growth potential, and Python's central role in their development ensures continued demand for Python skills well beyond 2025.
Python's Evolution: How the Language Stays Relevant
Recent and Upcoming Language Improvements
Python continues to evolve through regular updates, addressing previous limitations and adding new capabilities:
Performance Enhancements
Historically, Python has been criticized for its performance compared to languages like C++ or Java. However, significant improvements are addressing this limitation:
- The introduction of the mypyc compiler allows converting type-annotated Python code into optimized C extensions.
- Just-in-time compilation projects like PyPy continue to mature, offering substantial speed improvements for many applications.
- The Python core development team is actively working on performance optimizations, with each version showing incremental improvements.
By 2025, these efforts are expected to significantly narrow the performance gap between Python and traditionally faster languages for many common use cases.
Concurrency and Parallelism
Python's capabilities for concurrent and parallel programming continue to improve:
- The asyncio module has matured significantly, enabling efficient handling of thousands of concurrent connections in applications like web servers and microservices.
- Libraries like concurrent.futures simplify parallel execution, making better use of multi-core processors.
- Projects like Dask provide Python-native distributed computing capabilities for data-intensive applications.
These improvements address what was once considered a significant weakness in Python, making it more suitable for applications requiring high concurrency.
Type Hints and Static Analysis
Python's gradual typing system continues to evolve:
- Type hints, introduced in Python 3.5 and expanded in subsequent versions, enable better code documentation and error catching without sacrificing Python's dynamic nature.
- Static analysis tools like mypy, pyright, and pyre are becoming more sophisticated, catching potential errors before runtime.
- IDEs and code editors leverage these type hints to provide better auto-completion and refactoring support.
This evolution brings some of the benefits of statically-typed languages to Python while maintaining its flexibility and readability.
Community and Governance
Python's vibrant community and governance model contribute significantly to its continued relevance:
Open Governance Model
After creator Guido van Rossum stepped down as "Benevolent Dictator For Life" in 2018, Python adopted a more distributed governance model:
- The Python Steering Council ensures the language evolves in a way that balances innovation with stability.
- The Python Enhancement Proposal (PEP) process allows community members to propose and discuss language changes in a structured manner.
- Special interest groups focus on specific aspects of Python, from packaging to data science, ensuring the language meets the needs of different user communities.
This governance structure helps Python adapt to changing requirements while maintaining its core philosophy and backward compatibility.
Corporate Sponsorship and Investment
Major technology companies continue to invest heavily in Python:
- Google, Microsoft, Amazon, and Facebook employ core Python developers and contribute to language development.
- These companies also maintain important Python libraries and frameworks, ensuring they remain well-maintained and updated.
- Cloud providers offer optimized Python environments and services, further entrenching Python in enterprise environments.
This corporate backing provides resources for continued development and helps ensure Python's relevance in commercial applications.
Educational Focus
Python's position as the leading language for programming education strengthens its long-term prospects:
- Most universities and coding bootcamps use Python as an introductory programming language.
- Educational resources for Python are abundant, from free online tutorials to comprehensive courses.
- This educational focus creates a continuous pipeline of new Python developers entering the workforce.
As these students progress in their careers, they often bring Python with them, further expanding its adoption across industries.
Python 2 to 3 Transition Completion
The long transition from Python 2 to Python 3, once seen as a risk to Python's adoption, has been successfully completed:
- Support for Python 2 officially ended in 2020, unifying the community around Python 3.
- Virtually all major libraries and frameworks now support Python.
- This unified focus allows for faster innovation and improvement without the burden of maintaining compatibility with two major versions.
This successful transition demonstrates the community's ability to manage significant changes while maintaining Python's popularity and relevance.
Learning Python in 2025: Efficient Paths to Mastery
Modern Learning Approaches
The landscape of Python education continues to evolve, offering more efficient and effective learning paths for different goals:
Specialized Learning Tracks
Rather than generic Python courses, 2025 offers more specialized learning paths aligned with career goals:
- Data Science Track: Focused curriculum combining Python fundamentals with statistics, data manipulation, visualization, and machine learning.
- Web Development Track: Python basics followed by framework-specific training (Django, Flask, FastAPI) and database integration.
- Automation Track: Emphasis on scripting, system interaction, and workflow automation tools.
- AI/ML Track: Core Python leading to deep dives into neural networks, NLP, computer vision, and other ML specializations.
These specialized tracks enable learners to acquire relevant skills more efficiently than general-purpose Python courses.
Interactive Learning Platforms
Learning platforms continue to evolve beyond simple video courses:
- Notebooks-as-curriculum platforms integrate explanations, code execution, and visualization in a single environment.
- AI-assisted coding tutors provide personalized feedback and suggestions as learners write code.
- Virtual labs simulate real-world scenarios, allowing learners to apply Python to realistic problems.
- Gamified learning systems maintain engagement through achievement systems and progressive challenges.
These innovations make the learning process more engaging and effective, reducing the time needed to achieve proficiency.
Community-Driven Education
The Python community continues to play a crucial role in education:
- Mentorship programs connect beginners with experienced developers for personalized guidance.
- Code review communities provide feedback on learners' projects, accelerating skill development.
- Collaborative learning cohorts enable peer support and motivation through shared learning journeys.
- Open-source contribution pathways guide learners from simple documentation fixes to more complex code contributions.
These community resources complement formal education, providing practical experience and professional networking opportunities.
Essential Skills Beyond Core Python
To maximize career opportunities with Python in 2025, learners should develop complementary skills:
DevOps and Deployment
Understanding how to deploy and maintain Python applications is increasingly important:
- Containerization with Docker and orchestration with Kubernetes for scalable applications
- CI/CD pipelines for automated testing and deployment
- Infrastructure as Code using Python tools like Pulumi or AWS CDK
- Monitoring and observability for production Python applications
These skills enable developers to take applications from development to production, increasing their value to employers.
Database Knowledge
Data storage and retrieval remain critical skills:
- Relational databases (PostgreSQL, MySQL) and ORMs like SQLAlchemy
- NoSQL databases (MongoDB, Redis) and their Python interfaces
- Graph databases for connected data scenarios
- Time-series databases for metrics and monitoring applications
The ability to design efficient data models and write optimized queries complements Python development skills.
Cloud Services Integration
Cloud computing knowledge becomes increasingly inseparable from Python development:
- AWS, Azure, or Google Cloud Platform fundamentals
- Serverless Python deployment with cloud functions
- Cloud-native storage and database services
- API gateway and service integration
Experience with these services allows developers to leverage scalable infrastructure without managing servers.
Software Engineering Practices
Professional Python development requires solid engineering practices:
- Test-driven development and automated testing frameworks
- Code quality tools and linters
- Documentation best practices
- Design patterns and architecture principles
These practices distinguish professional developers from those who simply know the Python syntax.
Resources for Effective Learning
The ecosystem of Python learning resources continues to expand and improve:
Comprehensive Documentation
Python's official documentation remains exceptional, complemented by additional resources:
- The official Python tutorial and library reference
- Framework-specific documentation with interactive examples
- Community-maintained wikis and knowledge bases
- Annotated source code for popular libraries
These resources provide authoritative information directly from the developers and maintainers.
Practical Project Portfolios
Building a portfolio of practical projects accelerates skill development and demonstrates capabilities to employers:
- Guided project tutorials with increasing complexity
- Real-world problem repositories offering challenges based on actual scenarios
- Open-source contribution opportunities with mentorship
- Industry-specific project templates aligned with job requirements
These hands-on experiences provide both learning opportunities and portfolio material for job applications.
Continuous Learning Strategies
Given Python's ongoing evolution, strategies for staying current are essential:
- Following release notes and Python Enhancement Proposals (PEPs)
- Subscribing to Python-focused newsletters and podcasts
- Participating in virtual conferences and meetups
- Setting aside regular time for experimentation with new features and libraries
These habits ensure developers maintain current knowledge in a rapidly evolving ecosystem.
Industry-Specific Python Applications in 2025
Finance and Banking
Python's role in financial services continues to expand beyond its traditional use in quantitative analysis:
Algorithmic Trading and Quantitative Analysis
Python remains dominant in trading strategies and financial modeling:
- High-frequency trading systems using optimized Python with libraries like Numba
- Backtesting frameworks for evaluating trading strategies against historical data
- Risk management models for assessing portfolio exposure
- Sentiment analysis of financial news and social media for market prediction
These applications leverage Python's data processing capabilities and rich ecosystem of financial libraries.
Regulatory Compliance and Reporting
Financial institutions increasingly use Python for compliance:
- Automated regulatory reporting systems
- Transaction monitoring for fraud and money laundering detection
- Stress testing models for capital adequacy assessment
- Data validation pipelines ensuring reporting accuracy
Python's data manipulation capabilities and integration with database systems make it ideal for these applications.
Personal Finance and Banking Applications
Consumer-facing financial applications leverage Python:
- Budget management and financial planning tools
- Investment portfolio optimization
- Credit scoring and loan approval systems
- Personalized financial product recommendations
These applications combine financial algorithms with user-friendly interfaces, often using Python for the computational backend.
Healthcare and Life Sciences
Python's applications in healthcare continue to expand with the increasing digitization of medical information:
Medical Imaging and Diagnostics
Python powers advanced medical imaging applications:
- Diagnostic tools using computer vision to identify abnormalities in X-rays, MRIs, and CT scans
- 3D reconstruction of medical images for surgical planning
- Automated tissue classification and segmentation
- Quality assurance tools for medical imaging equipment
These applications leverage Python's computer vision and deep learning libraries to augment medical professionals' capabilities.
Genomics and Bioinformatics
Python is central to biological data analysis:
- Genome sequencing analysis pipelines
- Protein structure prediction
- Phylogenetic analysis and evolutionary studies
- Drug discovery and molecular modeling
Python's data processing capabilities and scientific libraries make it particularly suitable for these computation-intensive applications.
Clinical Trials and Research
Python streamlines medical research processes:
- Patient recruitment and matching for clinical trials
- Statistical analysis of trial results
- Natural language processing for medical literature review
- Adverse event detection and reporting
These applications improve research efficiency and help extract insights from complex medical data.
Manufacturing and Industry 4.0
The industrial sector increasingly adopts Python for automation and optimization:
Predictive Maintenance
Python enables condition-based maintenance strategies:
- Sensor data analysis to predict equipment failures
- Anomaly detection in machine operation
- Maintenance scheduling optimization
- Remaining useful life estimation for critical components
These applications help reduce downtime and maintenance costs through data-driven decision making.
Supply Chain Optimization
Python powers advanced supply chain analytics:
- Demand forecasting models
- Inventory optimization
- Logistics route planning
- Supplier performance analysis
These applications help manufacturers reduce costs and improve reliability throughout their supply chains.
Quality Control and Assurance
Python enhances quality management processes:
- Automated visual inspection using computer vision
- Statistical process control and quality metrics
- Defect prediction models
- Root cause analysis of quality issues
These applications improve product quality while reducing the need for manual inspection.
Environmental Science and Sustainability
Python plays an increasing role in addressing environmental challenges:
Climate Modeling and Analysis
Python facilitates climate research:
- Climate data analysis and visualization
- Predictive modeling of climate patterns
- Impact assessment of climate policies
- Integration of satellite and ground-based observations
These applications help scientists understand climate dynamics and inform policy decisions.
Resource Management
Python supports sustainable resource utilization:
- Smart grid management and energy optimization
- Water resource monitoring and conservation
- Precision agriculture for optimized crop yields
- Waste reduction and recycling process optimization
These applications help organizations reduce environmental impact while maintaining operational efficiency.
Environmental Monitoring
Python powers environmental monitoring systems:
- Air and water quality monitoring networks
- Wildlife population tracking and habitat analysis
- Deforestation and land use change detection
- Pollution source identification using sensor data
These applications provide timely information for environmental protection efforts.
Career Transition to Python: Strategies for Professionals
Pathways for Different Backgrounds
Python's accessibility makes it an excellent choice for career changers from various backgrounds:
For Non-Technical Professionals
Individuals from non-technical fields can leverage domain expertise while learning Python:
- Business and Finance: Start with data analysis and automation of business processes, then progress to financial modeling and reporting systems.
- Healthcare: Begin with healthcare data analysis, then advance to clinical decision support tools or medical imaging applications.
- Education: Start with educational content tools and grading automation, then move to learning analytics and personalized education systems.
- Marketing: Begin with marketing analytics and automation, then advance to customer segmentation and recommendation systems.
This approach allows career changers to apply Python to familiar problems while developing technical skills.
For Technical Non-Programmers
Professionals with technical backgrounds but limited programming experience can follow specialized paths:
- Database Administrators: Leverage existing database knowledge while learning Python for data extraction, transformation, and loading (ETL) processes.
- Network Engineers: Apply Python to network automation, monitoring, and configuration management.
- System Administrators: Start with scripting for system maintenance, then progress to infrastructure as code and cloud management.
- QA Specialists: Begin with test automation, then advance to building comprehensive testing frameworks.
These paths build on existing technical knowledge while adding Python programming capabilities.
For Developers in Other Languages
Experienced developers can transfer their skills to Python:
- Java/C# Developers: Focus on the differences in syntax and paradigms, while leveraging existing knowledge of object-oriented programming.
- JavaScript Developers: Emphasize server-side development with Python web frameworks and asynchronous programming models.
- R/MATLAB Users: Transition to Python's scientific stack (NumPy, Pandas, Matplotlib) while applying existing statistical knowledge.
- C/C++ Developers: Learn to appreciate Python's high-level abstractions while leveraging opportunities to integrate with existing C/C++ code for performance-critical sections.
These transitions capitalize on transferable programming concepts while adapting to Python's specific approaches.
Practical Transition Strategies
Successfully transitioning to Python development requires a structured approach:
Portfolio Development
Building a relevant portfolio demonstrates Python capabilities to potential employers:
- Create projects that solve problems relevant to your target industry
- Contribute to open-source Python projects in your area of interest
- Develop tools that showcase both domain knowledge and Python skills
- Document your learning journey through blog posts or technical articles
This portfolio provides tangible evidence of capabilities beyond certifications or course completions.
Networking in the Python Community
The Python community offers valuable support for career transitions:
- Join Python user groups and attend meetups (virtual or in-person)
- Participate in Python conferences like PyCon
- Engage in online communities like Python-specific Discord servers or Reddit's r/learnpython
- Connect with Python developers on LinkedIn and other professional networks
These connections provide mentorship, job leads, and insights into industry-specific Python applications.
Specialized Certification Paths
While certifications are less emphasized in Python than in some other fields, certain credentials can assist with career transitions:
- Cloud provider certifications that include Python (AWS Developer, Google Cloud Professional Data Engineer)
- Data science certifications that demonstrate Python skills in context
- Framework-specific certifications for Django, TensorFlow, or other specialized tools
- University or bootcamp credentials that demonstrate comprehensive Python knowledge
These certifications can be particularly valuable for career changers without formal computer science backgrounds.
Common Challenges and Solutions
Career transitions to Python development come with specific challenges:
Impostor Syndrome and Confidence Building
Many career changers struggle with confidence in their new skills:
- Start with small, achievable projects to build confidence
- Maintain a "learning log" documenting progress and achievements
- Find opportunities to explain concepts to others, reinforcing understanding
- Recognize that experienced developers also continuously learn and adapt
These approaches help build confidence through tangible accomplishments and perspective.
Bridging Knowledge Gaps
Career changers often have uneven knowledge distributions:
- Identify and systematically address fundamental computer science concepts that may be missing
- Join study groups focused on areas of weakness
- Use spaced repetition systems to reinforce learning in challenging areas
- Apply new concepts immediately in practical projects to strengthen understanding
This targeted approach addresses specific gaps rather than unnecessarily repeating familiar material.
Establishing Credibility
Demonstrating capabilities to potential employers can be challenging:
- Actively contribute to open-source projects to build a public track record
- Solve real problems for non-profits or community organizations
- Create detailed write-ups of projects explaining technical decisions
- Prepare for technical interviews with a focus on explaining thought processes
These strategies help demonstrate capabilities beyond formal credentials and work history.
Python's Economic Impact: Beyond Individual Careers
Organizational Benefits of Python Adoption
Beyond individual career opportunities, Python offers significant advantages to organizations:
Cost Efficiency
Python adoption often leads to cost savings:
- Reduced licensing costs due to Python's open-source nature
- Lower development time compared to more verbose languages
- Extensive library ecosystem that eliminates the need to build common functionality
- Easier maintenance due to Python's readability and simplicity
These factors contribute to lower total cost of ownership for Python-based solutions.
Workforce Flexibility
Python's accessibility creates workforce advantages:
- Wider talent pool due to Python's popularity
- Easier cross-training of existing staff
- Reduced onboarding time for new team members
- Facilitation of collaboration between technical and non-technical staff
These benefits improve organizational agility and resilience against talent shortages.
Innovation Acceleration
Python enables faster innovation cycles:
- Rapid prototyping capabilities for testing new ideas
- Easy integration with emerging technologies through Python libraries
- Lower barriers between research and production implementation
- Facilitation of cross-functional collaboration on innovative projects
These characteristics help organizations bring new ideas to market more quickly.
Python's Role in Digital Transformation
Python has become a key enabler for digital transformation initiatives:
Legacy System Modernization
Python helps organizations modernize their technology stack:
- Creating APIs around legacy systems
- Building data pipelines that extract value from historical data
- Developing modern interfaces for traditional backend systems
- Gradual replacement of legacy components with Python-based microservices
These approaches allow incremental transformation rather than risky "big bang" replacements.
Data-Driven Decision Making
Python enables the shift to data-driven organizations:
- Building internal analytics capabilities and dashboards
- Developing predictive models for business forecasting
- Creating recommendation systems for customer engagement
- Implementing anomaly detection for business processes
These applications help organizations leverage their data for competitive advantage.
Process Automation
Python drives automation across organizations:
- Robotic Process Automation (RPA) for routine tasks
- Workflow orchestration across disparate systems
- Document processing and information extraction
- Intelligent routing and processing of customer inquiries
These automation capabilities free human resources for higher-value activities.
Economic Sectors Transformed by Python
Python's impact extends across diverse economic sectors:
Retail and E-commerce
Python is reshaping retail experiences:
- Personalized recommendation engines
- Dynamic pricing optimization
- Inventory and supply chain management
- Fraud detection and prevention
These applications help retailers compete in an increasingly digital marketplace.
Transportation and Logistics
Python improves efficiency in moving goods and people:
- Route optimization algorithms
- Predictive maintenance for vehicle fleets
- Demand forecasting for capacity planning
- Real-time tracking and exception management
These capabilities reduce costs while improving service reliability.
Energy and Utilities
Python supports the evolution of energy systems:
- Smart grid optimization and management
- Renewable energy integration and forecasting
- Consumption pattern analysis and demand response
- Preventive maintenance for infrastructure
These applications contribute to more reliable and sustainable energy systems.
Agriculture
Python drives precision agriculture practices:
- Crop yield prediction models
- Automated irrigation systems
- Pest and disease detection using computer vision
- Soil quality analysis and fertilizer optimization
These technologies improve agricultural productivity while reducing environmental impact.
Ethical Considerations and Responsible Python Development
Privacy and Data Protection
As Python is frequently used for data processing applications, privacy considerations are paramount:
Privacy-Preserving Techniques
Python developers should implement privacy protections:
- Differential privacy implementations for sensitive data analysis
- Data minimization principles in application design
- Anonymization and pseudonymization techniques
- Privacy-by-design approaches in data pipelines
These practices help protect individual privacy while enabling valuable data analysis.
Compliance Frameworks
Python applications must meet evolving regulatory requirements:
- GDPR compliance for applications handling European data
- CCPA/CPRA considerations for California residents
- HIPAA requirements for healthcare applications
- Industry-specific regulations like GLBA for financial applications
Python developers need to understand these frameworks and implement appropriate safeguards.
Data Governance
Responsible Python development includes proper data governance:
- Metadata management for data lineage tracking
- Access control systems for sensitive information
- Data quality validation pipelines
- Retention and deletion policies implementation
These practices ensure responsible handling of data throughout its lifecycle.
AI Ethics and Algorithmic Bias
Python's prominence in AI development brings ethical responsibilities:
Bias Detection and Mitigation
Python developers should address algorithmic bias:
- Testing for discriminatory outcomes across demographic groups
- Implementing fairness metrics and constraints in algorithms
- Diversifying training data to reduce representation biases
- Conducting regular bias audits of deployed systems
These practices help prevent AI systems from perpetuating or amplifying societal biases.
Explainability and Transparency
Python AI applications should strive for transparency:
- Implementing model explanations using libraries like SHAP or LIME
- Documenting model limitations and appropriate use cases
- Providing confidence measures with predictions
- Creating user-friendly explanations of algorithmic decisions
These approaches help users understand and appropriately trust AI systems.
Human-in-the-Loop Systems
Responsible AI development often includes human oversight:
- Designing systems with appropriate human review points
- Implementing feedback mechanisms for correcting errors
- Creating override capabilities for edge cases
- Establishing clear escalation paths for disputed decisions
These designs balance automation benefits with necessary human judgment.
Environmental Impact
Python development has environmental implications that responsible developers should consider:
Efficient Computing
Python applications should minimize resource usage:
- Optimizing algorithms for computational efficiency
- Right-sizing cloud resources for actual needs
- Implementing caching strategies to reduce redundant computation
- Leveraging asynchronous processing to improve hardware utilization
These practices reduce the energy footprint of Python applications.
Green AI Practices
AI development in Python should consider environmental costs:
- Model architecture selection that balances accuracy with efficiency
- Transfer learning to reduce training energy requirements
- Model distillation for deploying lighter versions of complex models
- Carbon awareness in training scheduling
These approaches reduce the substantial energy footprint of advanced AI models.
Sustainability Applications
Python can positively impact sustainability efforts:
- Energy usage optimization applications
- Carbon footprint monitoring and reduction tools
- Resource conservation systems
- Environmental impact assessment automation
These applications demonstrate how Python skills can contribute to addressing environmental challenges.
Conclusion: Python's Enduring Value Proposition
As we've explored throughout this comprehensive guide, Python's position as a valuable and lucrative skill set continues to strengthen as we approach 2025. The language's remarkable versatility across domains—from web development to artificial intelligence, from data science to automation—ensures that Python proficiency opens doors to multiple career paths and creates opportunities in virtually every industry.
Python's sustained popularity isn't accidental; it stems from a powerful combination of technical capabilities, community strength, and real-world utility. The language continues to evolve, addressing historical limitations in performance and concurrency while maintaining the readability and accessibility that made it popular. The ecosystem of libraries and frameworks expands constantly, allowing Python developers to leverage existing solutions rather than building from scratch.
For individuals considering their career paths in 2025 and beyond, Python offers an exceptional blend of accessibility and depth. Beginners can quickly achieve productivity while having a clear path to mastery in specialized domains. Experienced developers from other languages can transfer their skills while accessing Python's unique strengths. Non-technical professionals can leverage Python as a gateway to technical roles, building on their domain expertise.
The economic case for Python skills remains compelling, with strong salary prospects across experience levels and specializations. Python developers enjoy both job security from consistent demand and career flexibility from Python's cross-domain applicability. Organizations benefit from Python adoption through cost efficiency, workforce flexibility, and accelerated innovation capabilities.
As with any technology, responsible Python development requires consideration of ethical implications, particularly in data privacy, algorithmic fairness, and environmental impact. The Python community increasingly emphasizes these considerations, developing best practices and tools to support ethical application development.
In conclusion, Python programming remains an excellent skill investment for 2025 and beyond. Its combination of technical capability, market demand, and ethical potential creates a unique value proposition for both individual careers and organizational success. Whether you're just beginning your programming journey or looking to expand your existing technical skills, Python offers a path to a rewarding and lucrative future in technology.
Big SALE:
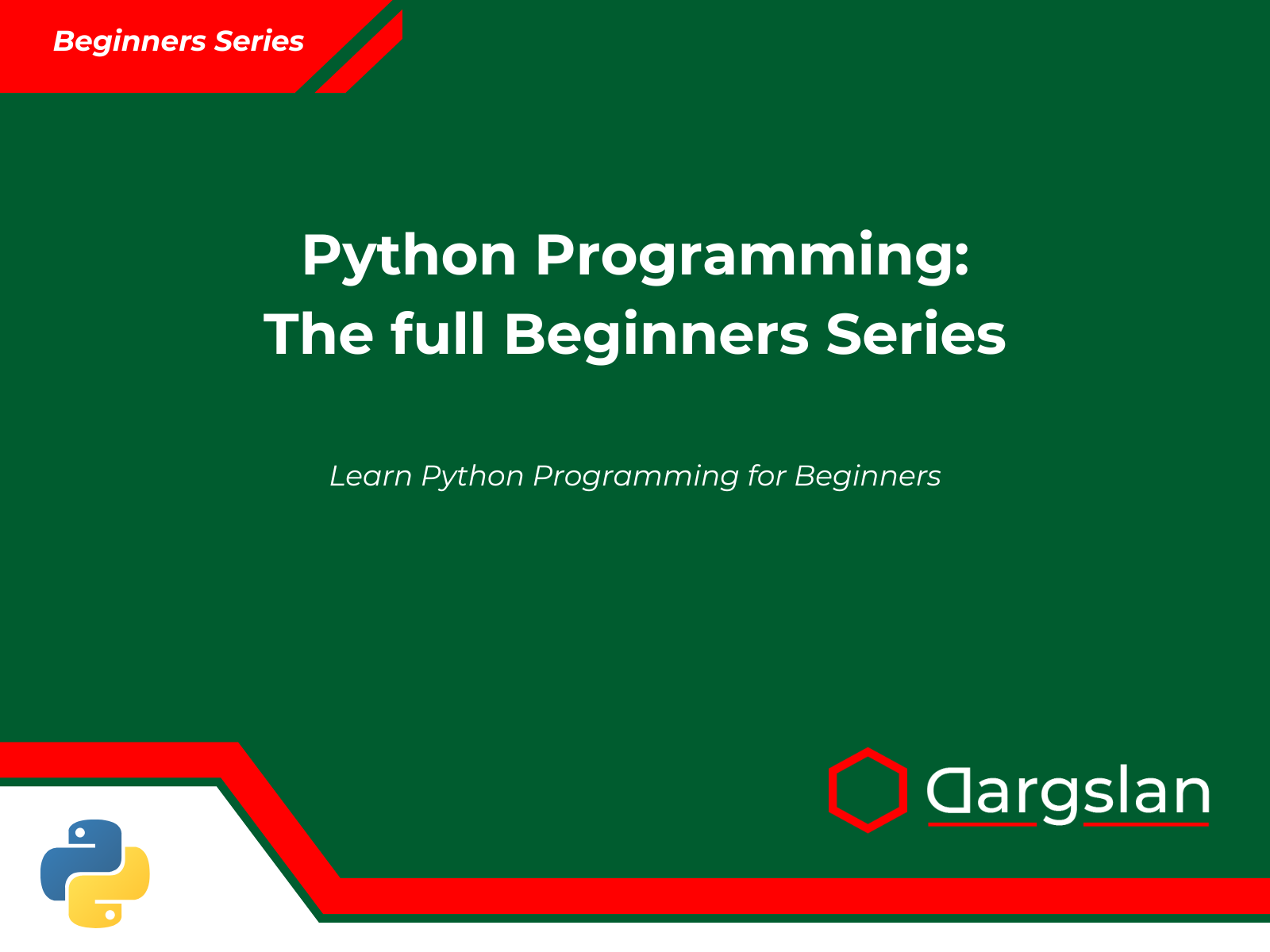